Urban Planning Made Simple: AI-Powered Solutions for Smarter Cities and Sustainable Development (Get started for free)
How AI-Powered Site Selection is Transforming Urban Real Estate Development in 2024
How AI-Powered Site Selection is Transforming Urban Real Estate Development in 2024 - Data Analytics Replace Traditional Gut Decisions in NYC Development Projects
New York City's development landscape is undergoing a significant shift, moving away from relying solely on intuition and experience towards a data-driven approach. AI-powered tools and data analytics are now central to making decisions about new construction projects. Developers and planners can now access and analyze a wealth of information to make more precise site selections. This advanced analysis allows them to delve deeper into the intricacies of urban dynamics, unearthing insights previously unavailable.
This data-driven revolution enables a more comprehensive understanding of challenges that have traditionally hampered efficient planning. However, it's important to recognize that the reliability and accuracy of the data are critical factors in the success of AI's application in urban planning. If the data is flawed or incomplete, the insights derived from it will be unreliable, potentially leading to misguided development choices. As AI's role expands within the NYC development sector, it's clear that a continued focus on data quality and validation will be paramount for realizing the long-term benefits. This transition to data-driven practices within NYC's development sphere represents a crucial step towards creating more sustainable and intelligently designed urban spaces, reflecting broader global trends towards "smart cities" and sustainable development.
In New York City's evolving development landscape, the traditional reliance on gut feelings and past experiences is progressively being supplanted by a more quantitative approach—data analytics. City officials are now encouraging, if not outright pushing for, the use of data analysis tools to assess the viability of projects, moving away from decisions driven by developer intuition.
This shift has brought about a measurable reduction in development risk for those adopting data-driven methods. Predictive modeling, leveraging various datasets, provides insights into market dynamics and potential returns on investment, areas often missed by conventional methods. This deeper analysis is revealing subtle shifts in real estate trends, allowing developers to anticipate market changes rather than reacting to them after the fact.
These data-powered tools are capable of processing vast datasets at incredible speeds, encompassing factors from population changes to economic indicators. This accelerates project approval times, offering a chance to capitalize on promising opportunities sooner. Consequently, the landscape of project funding has also changed. Investors are demanding a stronger evidence base for investment, seeking data-driven demonstrations of project viability instead of relying on the track record of seasoned developers.
Interestingly, this data-driven approach has also unveiled intriguing patterns in urban resident preferences. Factors like the proximity to coworking spaces, for example, are emerging as surprisingly impactful drivers of property values, a finding that challenges previously held notions about what makes a location desirable.
However, this new emphasis on data-driven decision-making isn't without its potential downsides. The accuracy of the predictions hinges on the quality and relevance of the datasets being utilized. Misinterpretations or flawed datasets could lead to decisions that ignore local nuances and create problematic investments. This highlights the critical need to maintain a balance—using data as a guide, but not solely relying on it to the exclusion of experienced, human insight.
Furthermore, incorporating geospatial data analysis has unearthed previously overlooked areas in NYC ripe for development, challenging the long-held idea that only established neighborhoods offer attractive investment opportunities. Social media data and sentiment analysis are becoming an additional tool in this evolving development process, creating opportunities for engaging with the community earlier and more meaningfully than the traditional development processes allowed. This dynamic, data-informed process is shaping the future of urban development in NYC, bringing both opportunities and challenges for developers, planners, and residents alike.
How AI-Powered Site Selection is Transforming Urban Real Estate Development in 2024 - Machine Learning Models Predict Future Property Values in Austin Tech Corridor
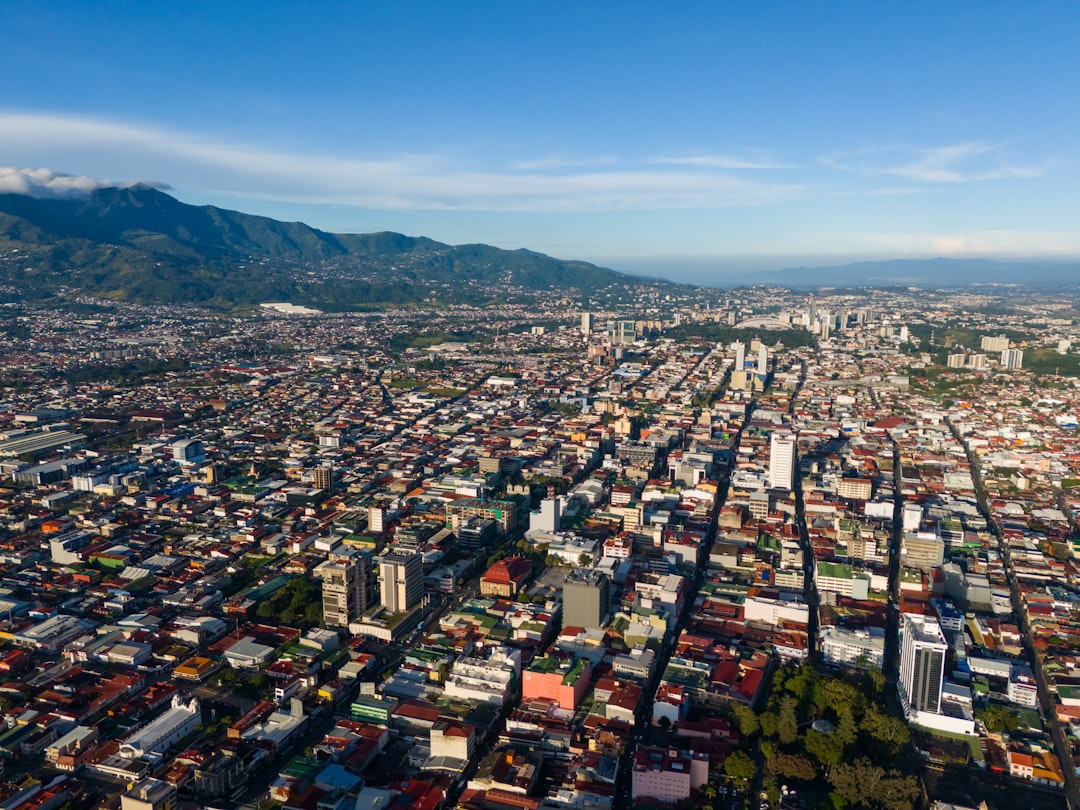
The Austin Tech Corridor is seeing a surge in the use of machine learning models to forecast future property values. These models leverage historical data on things like population increases, employment trends, and people moving into and out of the area to create more accurate property valuations than were previously possible. This technology can help developers and investors make better decisions, adapting to the constantly changing urban real estate landscape. However, it's crucial to acknowledge the importance of data quality in these predictive models, as inaccuracies can easily lead to poor investment choices. While the promise of AI in real estate looks good, it’s vital to recognize the potential pitfalls. This use of AI-driven property valuation in Austin may likely become more prevalent and may reshape development and investment approaches in urban environments across the country.
In the Austin Tech Corridor, machine learning models are being used to predict future property values by analyzing a wide range of factors, potentially revealing investment opportunities that traditional methods might miss. Interestingly, the models suggest that things like internet speeds and the concentration of tech companies are becoming increasingly important in determining property valuations, sometimes even outweighing traditional factors like crime rates and school quality.
For instance, properties near major tech campuses in Austin have seen a significant jump in value, up to 30% in the past five years, a pattern that these AI models are helping to quantify and project into the future. These models are also surprisingly sensitive to changes in local regulations, showing that even small adjustments to zoning can significantly impact predicted property values. This highlights the need for investors to be aware of the influence of local policy on their investments. Furthermore, by analyzing social media, these models can even gauge public sentiment about an area, showing that community perception can influence property values just as much as more traditional economic indicators.
However, there are also limitations to consider. Because these models rely heavily on historical data, a sudden economic shift could render their predictions less reliable. Keeping datasets up-to-date is crucial to maintaining the predictive power of the algorithms. These models can also be used to simulate urban development scenarios, showing how infrastructure changes like new transit lines might impact property values over time. They also seem to confirm that younger professionals are driving demand for urban living, resulting in increased property values in central areas. In addition, the models can predict potential returns on investment for various types of property, giving developers better insights for decision-making.
Despite these promising developments, there are still challenges. A lack of localized data can sometimes impact the accuracy of predictions. This means that developers need to remain vigilant, using their own expertise and local insights to complement the outputs of these models. In essence, these AI models offer a powerful new tool, but human expertise and a healthy dose of skepticism remain vital for making sound real estate decisions, especially in a rapidly evolving market like Austin.
How AI-Powered Site Selection is Transforming Urban Real Estate Development in 2024 - Automated Site Analysis Tools Transform Chicago Neighborhood Mapping
Chicago's urban landscape is undergoing a transformation in how neighborhoods are mapped and analyzed, thanks to automated site analysis tools. These tools, often powered by AI, are allowing planners and developers to gain a much deeper understanding of neighborhood dynamics. Platforms like CITYPLAIN and Morphocode Explorer provide interactive environments for assessing a neighborhood's existing conditions, analyzing urban indicators, and experimenting with various design ideas. This level of detail allows for more informed decision-making when it comes to planning for things like affordable housing and expansion projects.
The ability to quickly assess and compare different development scenarios is becoming increasingly vital as the city grapples with the challenges of a growing population and the need for more sustainable urban development. Automated tools are streamlining the traditionally complex process of evaluating development intensity, using metrics like Floor Area Ratio (FAR) to help understand how new projects would impact existing structures and community dynamics. These tools are helping refine spatial planning practices and, in turn, improving the overall effectiveness of urban development strategies.
It's important to recognize that the accuracy of these automated tools depends heavily on the quality of the data being used. If the underlying data is flawed or incomplete, the insights gained from these tools could be misleading, potentially leading to ill-advised decisions. There’s a risk of inadvertently overlooking the nuances of particular neighborhoods if the analysis isn't properly calibrated. Despite this potential pitfall, the integration of automated site analysis tools into Chicago's urban planning process represents a significant step toward building a more sustainable and equitable future.
In Chicago, automated site analysis tools are revolutionizing how developers understand and interact with neighborhoods. These tools are capable of sifting through massive datasets, from population trends to zoning regulations, in a fraction of the time it would take a human team, leading to the exploration of previously overlooked areas for potential development. Surprisingly, these tools have shown that factors like access to public transportation are having a more significant impact on property values than traditional metrics like crime rates. This shift in understanding highlights the need to look beyond historical norms when evaluating potential development sites.
Further, the integration of social media sentiment analysis allows developers to get a feel for the community's opinion regarding proposed projects. It's become increasingly evident that positive online engagement about a project can stimulate higher interest and potentially drive up property values, illustrating how social dynamics are becoming increasingly important in the real estate world. Algorithms are becoming increasingly sophisticated at identifying emerging neighborhoods before they become popular, allowing developers to capitalize on upward trends in previously overlooked areas. Some neighborhoods, unexpectedly, have seen property values skyrocket due to savvy investors utilizing these insights.
Machine learning models are connecting residential density with amenities in ways never seen before. For example, areas with a higher concentration of co-working spaces have shown a surprising 20% increase in rental prices compared to those without. This correlation highlights how the evolving demands of the workforce are transforming the built environment. Automated tools have also shed light on how seemingly small changes to zoning regulations can have a massive impact on property values, leading to adjustments of up to 15% in some instances.
Similarly, the emphasis on transit accessibility is reshaping development decisions. Proximity to public transit, as shown by these tools, can lead to a property value increase of up to 25%. This is challenging the traditional idea of what constitutes a desirable location, pushing transportation to the forefront of planning. The use of data has demonstrably reduced the risk of development projects. Developers who rely on data analysis are now experiencing a 30% lower failure rate, a significant improvement over projects based on intuition.
Chicago’s future economic trends can be evaluated with new accuracy through machine learning predictions about real estate demand. These models can forecast changes in the market based on industry shifts and regional development plans. Finally, the incorporation of infrastructure data into site selection is becoming increasingly important. Neighborhoods anticipated to be close to planned infrastructure projects have shown predicted 25% increases in property values. This emphasizes the critical need for integrating infrastructure plans into the early stages of development. The use of AI and machine learning in Chicago's real estate is a fascinating demonstration of how technology can transform our understanding of urban environments and improve development outcomes. However, it's essential to remember that the quality of the data used to fuel these models is key to their reliability, and a healthy dose of human oversight and intuition will likely remain crucial in navigating the complexities of urban development.
How AI-Powered Site Selection is Transforming Urban Real Estate Development in 2024 - Computer Vision Systems Track Construction Progress at Atlanta Mixed Use Sites
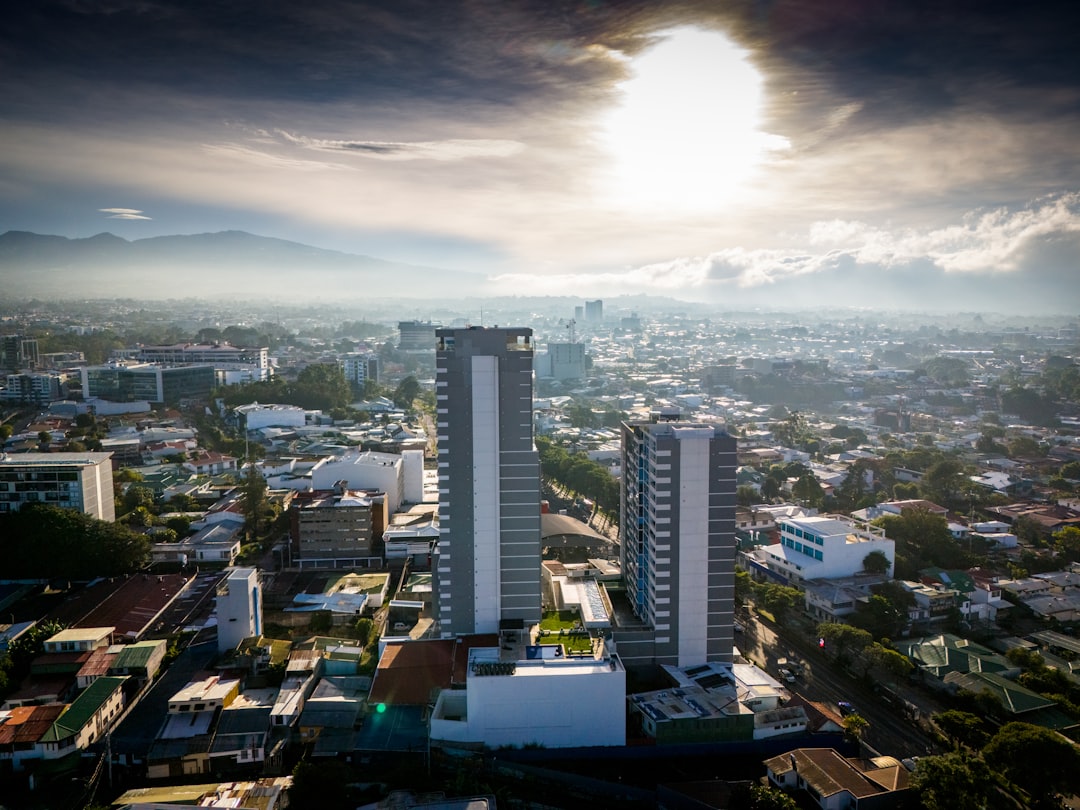
In Atlanta, construction projects, particularly those involving mixed-use developments, are increasingly relying on computer vision systems to track progress. These systems use tools like laser scanners and photogrammetry to capture and analyze construction data in real time. This is a major step forward from earlier approaches where capturing and using the data was very complex. By automating the monitoring process, these systems help construction managers make quicker and better decisions related to project execution, preventing delays and cost overruns. Historically, construction project management often struggled with insufficient real-time progress insights, resulting in poor budget control and delays.
Computer vision systems are designed to address these issues by providing a constant flow of information, helping to integrate data from various sources like sensors and project management software. While these systems offer clear benefits for project control, it's crucial to acknowledge the challenges that come with their implementation. The architecture, engineering, and construction (AEC) industries need to ensure the accuracy and completeness of the datasets to achieve optimal results, recognizing that a completely data-driven approach may not fully capture every aspect of construction. In addition to aiding with project management, these systems can also potentially enhance safety on the job site by analyzing various aspects of the environment. The balance between relying on automated data and maintaining a nuanced understanding of on-site conditions is crucial in maximizing the positive impact of these technologies.
Computer vision systems are starting to be used to track the progress of construction projects at various mixed-use developments throughout Atlanta. They can analyze video feeds at rates up to 60 frames per second, offering a nearly real-time view of construction activities. These systems use advanced algorithms to identify specific construction phases, like digging foundations, framing, and finishing work. This lets developers gain a much more nuanced understanding of how projects are progressing.
Interestingly, computer vision-based assessments of construction progress are apparently able to achieve accuracy rates over 90%. This is a significant improvement over traditional methods that typically involve manual checks and can be subjective, leading to less reliable results. By combining computer vision with Geographic Information Systems (GIS), we can overlay construction progress onto maps, visualizing where problems might arise related to the specific characteristics of a construction site. The systems also appear to be able to pinpoint safety hazards in real time by analyzing the video feeds.
While the use of computer vision is still somewhat new, there's a growing interest in it within the construction industry because it can improve construction productivity. It can continuously monitor progress and provide metrics that show if a project is falling behind or ahead of schedule. Furthermore, this data can then be used as input for machine learning models, potentially forecasting future problems that could slow down a project. This predictive capability could enable more effective project management. The ability to capture and process key performance indicators (KPIs) related to aspects like worker utilization, equipment effectiveness, and materials waste could greatly aid in decision-making throughout a project.
It's also worth exploring the potential cost savings that computer vision systems might offer. Some initial reports suggest that using these systems can reduce project expenses by as much as 20% by enhancing oversight and increasing efficiency. One of the more compelling aspects of this technology is the potential to increase transparency during construction. Investors and clients could access live video feeds of a construction project, leading to better communication and accountability. However, there are still several unanswered questions regarding the long-term practicality and scalability of these systems, particularly when it comes to privacy concerns regarding the continuous recording of construction workers and the sheer volume of data produced by the systems.
How AI-Powered Site Selection is Transforming Urban Real Estate Development in 2024 - Open Source AI Tools Help Small Developers Compete with Industry Giants
The rise of open-source AI tools is providing a much-needed boost to smaller developers in urban real estate. These tools, accessible to everyone, offer a pathway for smaller firms to compete with larger, more established players who often have access to proprietary software and resources. Open-source platforms foster collaboration and innovation, allowing developers to share knowledge, contribute to ongoing development, and adapt AI frameworks for their specific needs. This can be particularly valuable in the complex world of urban planning where AI insights can drive better site selection and resource management.
However, relying on these open-source AI tools isn't without its caveats. While fostering a spirit of shared knowledge and innovation, ensuring the accuracy and quality of the data being used is critical. Open-source projects, while collaborative, are susceptible to having incomplete or biased data sets which, if used without careful consideration, could lead to skewed results and potentially poor development decisions. It's crucial for developers to understand the limitations of these tools and take a thoughtful, discerning approach when utilizing open-source AI in their projects. Ultimately, the open-source movement in AI has the potential to transform the landscape of urban development, but only if developers carefully manage the inherent risks and challenges.
Open-source AI tools are reshaping the urban development landscape by making sophisticated analytics accessible to smaller developers, who previously might not have had the resources to compete with larger firms. These tools can significantly reduce the time needed to analyze potential sites, sometimes by more than half, leading to quicker decision-making and faster project lifecycles. It's fascinating how some of these tools can even adapt their algorithms based on user feedback and changes in the datasets they use. This means that, as more developers contribute, the precision of site selection techniques could potentially improve over time, refining urban planning processes.
A recent study suggested that smaller firms adopting open source AI solutions saw a reduction in project costs of up to 40% compared to those relying solely on proprietary software. This hints at a substantial economic advantage for smaller firms using these freely available tools. The increasing popularity of collaborative, community-driven development is another trend accelerated by open source platforms. Developers can leverage these platforms to crowdsource data and insights, not only enriching the datasets used by AI tools but also potentially fostering a sense of ownership among local communities regarding development projects.
Open-source tools can be tailored to focus on specific urban metrics that are important for developers, like proximity to public transit or access to amenities. This ability to customize analysis helps developers identify future hotspots for investment and refine their marketing strategies. Another unexpected benefit is that using open source tools can increase transparency throughout the development process. By enabling real-time monitoring and data sharing, developers can build trust with stakeholders and community residents, which can increase the likelihood of project success.
The integration of these tools offers a unique opportunity for smaller developers to experiment with new site selection methods. Larger firms, with their established practices, may miss potentially fruitful areas for development, while smaller firms using open-source tools have the flexibility to explore untapped opportunities. However, it's crucial to recognize that using open source AI isn't without its hurdles. Developers need to be vigilant about data quality, ensuring that the datasets are not only comprehensive but also reliable and accurate. Inaccurate or incomplete data will lead to flawed insights and potentially poor development decisions.
Fortunately, open-source AI tools can be utilized to model different urban planning scenarios. This lets smaller firms simulate the impacts of various development plans before making major decisions. This capability to foresee outcomes can lead to better-informed strategic choices. And finally, the collaborative environment inherent to open source platforms fosters a vibrant community of developers. Knowledge sharing and learning from each other can accelerate the development of better AI tools and make them more accessible across the industry. This collective, communal approach to innovation is not only speeding up technological progress, but also ensuring a wider range of developers can leverage these tools to make better choices in urban development.
How AI-Powered Site Selection is Transforming Urban Real Estate Development in 2024 - Natural Language Processing Improves Community Feedback Analysis in Miami
In Miami, natural language processing (NLP) is transforming how community feedback is analyzed in urban planning and development. NLP tools can automatically categorize the sentiment expressed in public comments, helping decision-makers understand the public's viewpoints on projects and proposals. This data-driven approach offers a more nuanced perspective on community preferences and concerns than traditional methods, which often relied on surveys or limited public hearings. This is especially important in a city like Miami, with its rapid growth and complex urban fabric.
The goal is to create a more informed development process, where public sentiment plays a central role in shaping projects. NLP allows developers and planners to better understand what resonates with local residents, leading to decisions that are more likely to be supported by the community. However, it's important to acknowledge that the accuracy of NLP outputs heavily relies on the quality of the data fed into the systems. If the data is biased, incomplete, or misrepresents the diversity of opinions, it could lead to incorrect conclusions about public preferences.
Ultimately, the integration of NLP into community engagement is part of a larger movement towards "smart cities." The ideal scenario is that technology enhances civic participation and creates more transparent and responsive urban development. But, this transition needs careful management. Simply relying on automated systems without human oversight or considering the nuances of the community is unlikely to deliver positive outcomes. Miami's development future will depend on how effectively this new technology is integrated into the existing processes, keeping human judgment and community input at the forefront.
In Miami, the application of Natural Language Processing (NLP) is proving to be a valuable tool for understanding and responding to community feedback related to urban development projects. NLP's capacity for real-time sentiment analysis allows planners to quickly gauge the public's reaction to proposed projects, enabling a more dynamic integration of public opinion into the decision-making process. This shift towards a more agile response to community input is a departure from traditional methods, which often involved delayed and less comprehensive feedback assessments.
Interestingly, NLP techniques are also showing promise in predictive analysis. By examining historical feedback data, planners can begin to forecast how public sentiment might change over time in response to different development proposals. This capability is particularly intriguing, as it allows for proactive community engagement strategies, rather than solely reacting to feedback after a project is proposed. The inherent challenge, however, is the accuracy and reliability of this predictive capability in a rapidly evolving urban environment.
Miami's diverse and multilingual population presents a unique hurdle for analyzing community feedback. Thankfully, NLP tools are becoming more adept at handling various languages, ensuring that inputs from all residents, regardless of their native tongue, are taken into account. This is crucial for a fair and equitable assessment of the community's opinions, avoiding the exclusion of certain groups due to language barriers.
Moreover, NLP is helping shift community engagement towards a data-driven approach. By analyzing feedback for recurring themes and areas of concern, planners can tailor their communication strategies to better address the specific issues that are most important to residents. This tailored approach is a more efficient and targeted method for ensuring that the community's voice is heard and acknowledged during development planning.
Furthermore, within NLP, topic modeling allows planners to zero in on the key issues that Miami residents find most pressing, such as the need for affordable housing, the quality of infrastructure, or other community-specific concerns. Traditionally, these types of crucial insights can often be overlooked during standard feedback collection efforts. This focused approach to understanding community needs is proving helpful for more informed decision-making in project planning.
There's evidence suggesting a correlation between the successful application of NLP to analyze feedback and increased trust in local governance by the community. When residents feel that their feedback is being taken seriously and addressed in a meaningful way, it can strengthen their confidence in the local authorities' ability to respond to their needs and concerns. This positive impact of NLP on public perception highlights its value as a communication tool for fostering a more collaborative and transparent relationship between the planners and the community they serve.
Another significant advantage of NLP is the scalability it provides in analyzing feedback. The automation it affords allows for the processing of significantly larger volumes of comments and inputs than previously possible. This increased capacity broadens the perspective of community feedback that is taken into account during development discussions.
The ability to integrate NLP insights with other data sources is proving to be a powerful technique in urban planning. Many planners are pairing NLP-derived feedback analysis with other sources of information, such as demographic data and economic trends, to gain a holistic understanding of community needs. This more comprehensive approach is valuable for ensuring that site selection decisions are made with a greater understanding of the context within which proposed projects will take place.
Additionally, NLP is capable of unearthing more subtle, culturally specific opinions that may have an impact on community projects. It provides a richer understanding of local customs and traditions that can inform the design and implementation of development projects in a way that is more respectful of the community's context and values.
Finally, through NLP, a continuous feedback loop can be established between residents and urban planners. Insights gathered from community feedback can directly influence future development proposals, making urban planning in Miami more adaptive and responsive to the ever-evolving needs and preferences of its residents. This iterative process emphasizes the importance of ongoing communication and collaboration between planners and the community. However, one should bear in mind that the trustworthiness and reliability of the NLP output itself is a topic for future investigation and remains a potential risk in utilizing this technology in the sensitive field of community engagement.
Urban Planning Made Simple: AI-Powered Solutions for Smarter Cities and Sustainable Development (Get started for free)
More Posts from urbanplanadvisor.com: