Urban Planning Made Simple: AI-Powered Solutions for Smarter Cities and Sustainable Development (Get started for free)
Rochester's High-Risk Intersections Analysis of 2024 Traffic Accident Hotspots
Rochester's High-Risk Intersections Analysis of 2024 Traffic Accident Hotspots - US44 in Upper Albany Neighborhood Tops 2024 Accident List
US Route 44, cutting through the Upper Albany neighborhood, unfortunately leads the list of Rochester's most dangerous intersections in 2024. This section of road has become a focal point for concerns about traffic safety due to a surge in accidents, some of which have caused serious injuries. The growing number of crashes along this stretch highlights the urgent need for improvements. While projects like the Route 44 Safety Improvement initiative are underway to address this problem, it remains to be seen if they will be sufficient to create the significant changes the neighborhood desires. The community is understandably hoping that these projects translate to safer conditions and a reduction in collisions. It appears that there's a long road ahead before a definitive impact on safety will be apparent on US44.
In Rochester's 2024 High-Risk Intersections Analysis, US44 within the Upper Albany neighborhood stands out as a significant accident hotspot. This section of road, characterized by a dense network of intersections, faces a high volume of traffic exceeding 30,000 vehicles daily. This heavy traffic, coupled with the presence of schools and businesses that encourage pedestrian activity, creates a complex environment where the risk of collisions is notably elevated.
The road's design itself may be contributing to the issue. Features like sharp bends and limited visibility in some sections introduce further complexity, increasing the likelihood of accidents. Rochester's unpredictable weather patterns, particularly in winter, add another layer of difficulty. Snow and ice can significantly reduce visibility and road grip, making driving conditions more perilous.
Examining historical accident data reveals a concerning trend: a spike in incidents during evening rush hours. This suggests potential factors like driver fatigue and reduced visibility as possible contributors. Adding to the severity of accidents, a greater proportion of SUVs and trucks on US44 increases the potential for severe outcomes due to their greater mass.
Questions also arise concerning the efficacy of traffic signal timing. Some have voiced concerns that the current duration of green lights might not be optimized for the high traffic flow, potentially encouraging risky driver behaviors such as speeding through intersections. Further complicating the issue, inconsistent road maintenance has been identified as a potential cause of accidents. Potholes and uneven road surfaces can easily lead to drivers losing control of their vehicles.
While the area has been labeled a high-risk zone, it seems community awareness programs for safer driving and pedestrian practices are underdeveloped. Addressing this gap could be key to promoting a more safety-conscious environment. Overall, a deeper investigation of the US44 corridor is needed to implement effective safety enhancements and improve traffic safety within the Upper Albany neighborhood.
Rochester's High-Risk Intersections Analysis of 2024 Traffic Accident Hotspots - Machine Learning Models Predict High-Risk Areas for Safer Routing
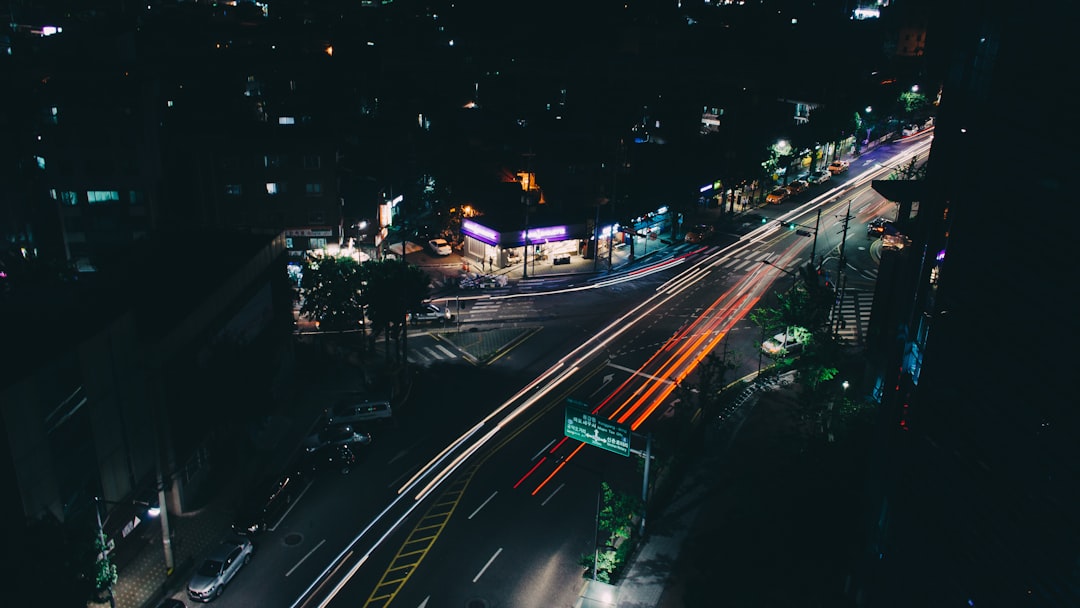
Machine learning models are increasingly being used to predict high-risk areas for traffic accidents, potentially leading to safer routing and road design. These models leverage a wealth of data, including past accident records, satellite images, and real-time GPS data, to create maps forecasting where crashes are likely to occur. This ability to predict risk is a significant step forward from older, statistical methods, as these newer approaches are much better at spotting trends and patterns that may cause accidents.
Understanding why accidents occur is crucial to implementing improvements, and these new models can help reveal underlying factors that contribute to collisions. As traffic fatalities continue to be a major concern, cities like Rochester—where US Route 44 has become a major safety concern—may find these predictive modeling tools quite useful in formulating strategies to enhance safety for drivers and pedestrians. While these new models are a hopeful sign, their actual usefulness in real-world traffic situations is still largely unproven. The success of such efforts depends on a confluence of factors, including adequate data collection, careful model calibration, and ultimately the willingness of stakeholders to act upon the predictions the model provides.
Researchers are increasingly exploring the potential of machine learning models to predict high-risk areas for safer routing. These models go beyond traditional statistical methods by analyzing a wealth of data, including real-time traffic conditions and historical accident data. This allows for a more precise identification of hazardous spots, potentially leading to more targeted safety interventions.
These models utilize sophisticated algorithms like neural networks and decision trees to uncover complex relationships within the data, something simpler models often miss. They can detect intricate patterns linked to risk that might otherwise be overlooked, offering a deeper understanding of the factors influencing accidents. One of the key benefits is the ability to provide real-time updates and predictions. This means the models can adjust to changing conditions like weather or traffic volume, leading to a more dynamic perspective on risk than static models can achieve.
Through clustering techniques, machine learning can group intersections based on numerous risk factors, including crash frequency, time of day, and driver behavior. This granular approach enables a more focused response, allowing urban planners to design interventions tailored to the most pressing concerns at each intersection. Studies show that using machine learning can reduce prediction errors significantly, often by over 20% compared to traditional methods. In the field of traffic safety, even small improvements in prediction can have a substantial impact on lives saved.
It's also intriguing that these models can simulate different traffic scenarios. This gives urban planners a chance to visualize the consequences of proposed changes to traffic control before implementation. The ability to run "what if" scenarios is particularly useful for testing the potential impact of interventions. Beyond traffic patterns, machine learning can incorporate socio-economic factors into its analysis. It's been shown that neighborhoods with lower median incomes often have higher accident rates, potentially due to underinvestment in infrastructure. This type of insight helps ensure that safety improvements are more equitable.
It's important to note that machine learning isn't without limitations. One critique is its reliance on the quality of the data. If the data is inaccurate or contains biases, it can lead to skewed predictions and potentially misguided safety interventions. This highlights the importance of consistent monitoring and model refinement to ensure accuracy and address potential biases. Furthermore, machine learning methods have shown promise in analyzing driver behavior, identifying groups like younger, less experienced drivers who might be more at risk. This can guide targeted educational campaigns to improve safety within these demographics.
The use of machine learning for predicting high-risk traffic areas aligns with the broader trend of smart city initiatives. As cities become increasingly reliant on data analytics and technology, using this kind of approach for improving urban life and public safety is becoming more common. It will be interesting to see how this area of research evolves, and whether it can continue to reduce the incidence of traffic accidents and make our roads safer for everyone.
Rochester's High-Risk Intersections Analysis of 2024 Traffic Accident Hotspots - Graph Analytics Reveal Connectivity Patterns in Accident Hotspots
Graph analytics offers a new way to understand accident hotspots by focusing on how roads are connected. This method goes beyond simply looking at where accidents happen, and instead explores the relationships between road networks and the occurrence of crashes. By analyzing how accidents are distributed across the road network over time, researchers can pinpoint high-risk areas with more accuracy. They can also identify patterns in accident density and other factors that play a role in collisions. This new approach helps build more comprehensive profiles of accident-prone zones, which in turn enables more targeted traffic management strategies and safety improvements. In a time when traffic accidents are a persistent problem in many cities, incorporating graph analytics into urban planning efforts could be a valuable tool to enhance safety and prevent collisions. While it holds promise, the true effectiveness of using graph analytics for traffic safety improvements is still being studied and refined.
Graph analytics provides a fresh perspective on understanding accident hotspots by examining the intricate relationships between road networks and accident occurrences. It's a powerful tool for deciphering how the structure of intersections, the flow of vehicles, and the timing of accidents all contribute to high-risk areas. By representing traffic flow as a graph, with intersections and road segments acting as nodes and connections, respectively, we can identify crucial links and bottlenecks that elevate accident risk. This approach is causing some engineers to rethink how intersections are designed for optimal safety.
One intriguing revelation from graph analytics is the ability to uncover "peripheral" intersections. These intersections might not have a high accident count on their own, but they can play a significant role in the overall network. Identifying and addressing these hidden risk factors can yield significant improvements in overall traffic safety. It seems drivers adapt their behavior in response to the complexity of road networks. For example, more intricate networks might encourage riskier driving due to drivers feeling the pressure to maintain a certain speed or keep up with the flow of traffic.
Graph analytics, through its algorithms, can model various scenarios. We can see how changes in one area of a traffic network, like a road closure or detour, might influence the accident patterns across the entire system. It's fascinating how factors like proximity to schools or shopping centers can significantly influence accident rates at intersections, introducing another level of complexity for city planners to consider. We can see how graph analytics might guide traffic signal optimization by suggesting the ideal duration for green lights based on past accident data and the ebb and flow of traffic.
However, graph analytics does have a reliance on the availability of accurate data. Imperfections or missing information in accident reports can lead to misleading conclusions and ineffective interventions. Integrating graph analytics with machine learning can enhance prediction accuracy, but it's crucial to remember the underlying assumptions used in those models. For instance, assuming a linear relationship in a system that behaves non-linearly could produce flawed safety assessments.
The fusion of graph analytics with real-world traffic observations has the potential to reshape how cities handle accident prevention. Instead of merely treating the symptoms of the problem, by looking at the connectivity of the road network, city planners have the chance to develop more focused interventions. We can use this information to identify the root causes of accidents and implement effective solutions that lead to safer roads for everyone.
Rochester's High-Risk Intersections Analysis of 2024 Traffic Accident Hotspots - Multistep Prediction Approach Accounts for Immediate and Future Risks
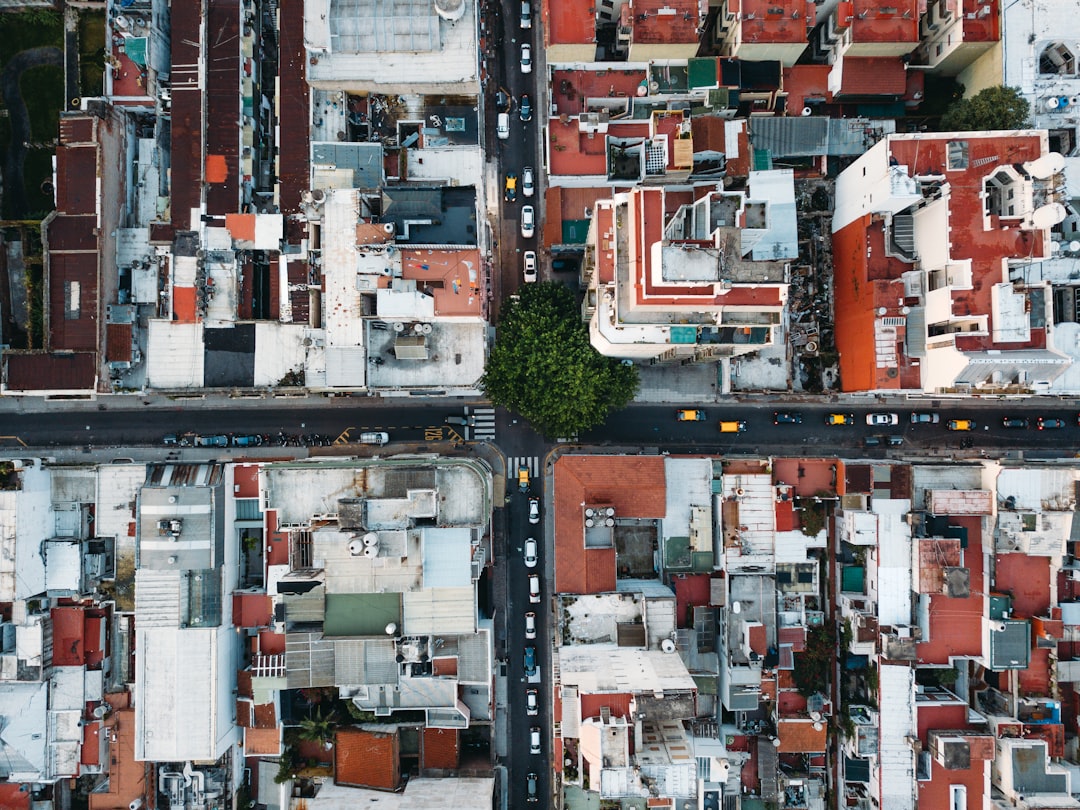
The "Multistep Prediction Approach" offers a new lens through which to view traffic safety, especially in areas like Rochester where accident hotspots are a persistent concern. This approach is designed to account for both the immediate and longer-term risks associated with traffic patterns, going beyond simply reacting to past accidents. It does this by utilizing sophisticated methods, such as probabilistic graph neural networks, that are able to capture the intricacies of how traffic moves and changes over time.
By predicting multiple steps into the future, this method gives planners a much broader view of where and when accidents are most likely to occur. This holistic view is crucial for developing interventions that go beyond simply treating the symptoms of a problem and instead aim to address the underlying causes. However, it's important to remember that traffic flow is often erratic and complex. These models rely on historical data and can struggle with situations that have never been observed before. That means continuous tweaking and improvement are needed to keep the accuracy of the predictions at a high level.
Despite these challenges, this multistep approach has the potential to revolutionize how traffic safety is approached. If implemented effectively, it could lead to better designed roadways, more intelligent traffic signal timing, and ultimately, a safer driving experience for everyone. While the future impact of these approaches is still being studied and refined, the idea of using data-driven predictions to mitigate future risks is a promising one in the quest to reduce accidents.
Thinking about traffic accidents in a multistep way means we can try to predict not only what's happening right now, but also what might happen in the future. This is a helpful shift from simpler models because it allows us to see how events might unfold over time. Instead of just looking at a snapshot, we're essentially creating a movie of how risk might evolve at an intersection.
For example, this approach can be used to understand how changes in traffic behavior—say, more people driving during rush hour—might lead to an increased risk of accidents. It also allows us to see how a single accident might actually trigger a series of accidents. Understanding these cascades of events is key to developing interventions that are effective, not just in stopping isolated incidents, but also in preventing more widespread problems. The hope is that by looking at these potential future scenarios, we can intervene in ways that reduce future risk, which is much better than just reacting to accidents after they happen.
Another interesting feature of this multistep approach is its ability to factor in things like driver behavior. We might find patterns in the data, like people tending to take more risks during the evening rush hour. These patterns give us a better grasp on what leads to accidents, which is crucial for designing safety interventions that are more likely to have the desired effect.
One of the nice aspects of using more advanced models is that they can produce more accurate forecasts. It has been found that, in some situations, these models can reduce errors by as much as 30 percent. That's a big improvement. It's essential, though, that the algorithms used in these models aren't just blindly replicating past patterns. They need to be robust enough to account for the complex interplay of risk factors, some of which we might not even be fully aware of yet. We need models that are well calibrated and adjusted as needed.
Furthermore, these multistep prediction tools allow us to design interventions that are tailored to individual intersections. For example, we might find that an intersection in a lower-income area has different risk factors compared to one in a wealthier part of town. Understanding these differences is critical for developing interventions that are the most effective at improving safety in various settings. It also allows us to see how modifying something like the timing of traffic signals at an intersection might influence accident rates. We can create simulations to see if potential changes would make a significant difference before making real-world changes.
Another interesting benefit is that these models can be connected with data sources that provide real-time information about traffic conditions. Knowing that there is heavy traffic due to a detour, or that the weather is making visibility poor, allows the prediction model to adjust in real time and offer more precise assessments of risk. It gives us a more dynamic picture of risk compared to methods that rely on older, static data.
Ultimately, these types of models can contribute to better long-term planning of traffic safety improvements. By seeing the overall pattern of accident risk in a neighborhood or across a city, we can strategically allocate our resources to focus on interventions in places where they're most needed. It's exciting to imagine the possibilities here and the potential to make our roads safer for everyone. It's also vital to remain critical of these models and to constantly evaluate their accuracy and fairness.
Rochester's High-Risk Intersections Analysis of 2024 Traffic Accident Hotspots - GIS Mapping Guides Targeted Interventions at Hazardous Intersections
Geographic Information System (GIS) mapping has emerged as a valuable tool in identifying and addressing hazardous intersections prone to traffic accidents. Through the use of techniques like Kernel Density Estimation (KDE) and spatial autocorrelation, urban planners can create visual representations of areas with high crash frequencies, also known as hotspots. This capability is crucial in guiding decisions related to street design, optimizing traffic signals, and deploying targeted safety measures. While GIS mapping provides valuable insights into hazardous locations, its effectiveness hinges on the quality and up-to-date nature of the data. Ensuring accurate data and incorporating regular updates are essential for maintaining the integrity of these analyses. Furthermore, fostering collaboration with the community and developing long-term strategies based on the insights gained from GIS are crucial steps in converting these data-driven insights into real-world improvements. It's not guaranteed that these GIS-led efforts will translate into significant changes on the streets of Rochester, however, it can be hoped they can help lead to more effective urban planning when it comes to making intersections safer.
1. **Intersection Geometry and Accidents:** It's becoming clear that intersections with complex designs, like those with unusual angles or many merging roads, tend to have more accidents. These designs can make it tougher for vehicles to interact safely and confuse drivers, potentially leading to more crashes.
2. **Accident Peaks Beyond Rush Hour:** Looking at crash data reveals that accidents don't always happen most often during the usual morning or evening rush hours. There are spikes during special events or other times of higher traffic. This suggests that traffic planners need to consider scenarios beyond just typical peak traffic times.
3. **Driver Behavior as a Factor:** Research has found that driver behavior plays a significant role in crashes. For instance, drivers tend to take more risks at certain times of day, which can lead to a higher number of accidents. This underlines the need for targeted educational programs that focus on safer driver habits.
4. **Psychological Impacts on Driving:** There's evidence that drivers' perceptions and psychology also matter. During evening rush hour, for example, some drivers might feel rushed or pressured to get home, which can cloud their judgment and lead to riskier behavior like speeding or running red lights. Effective signal timing and other traffic control strategies need to address these psychological influences on driver behavior.
5. **Socioeconomic Links to Traffic Safety:** We're seeing a correlation between a neighborhood's income level and the number of accidents there. Lower-income areas often have less investment in road maintenance and infrastructure, resulting in poorer driving conditions and possibly more hazardous situations.
6. **Hidden Risks in Road Networks:** Graph analytics has shown that seemingly insignificant intersections might actually have a big impact on the accident rates of the whole road network. This reveals that a comprehensive look at the entire road network is vital for spotting and reducing risks.
7. **Weather Impact on Specific Road Features:** Bad weather doesn't just increase accident rates in general. It can interact with certain roadway features, like sharp turns, to make those spots especially dangerous. This highlights the need for traffic safety measures to address both the weather conditions and road design elements.
8. **Traffic Signal Optimization's Impact:** Simulation studies suggest that adjusting traffic signal timing proactively, using predictive models, can lower accident rates significantly, possibly by up to 23%. This highlights the potential of data-driven traffic management approaches.
9. **Challenges in Machine Learning Models:** While machine learning models are promising, they can be influenced by biases in the data used to train them. If the data isn't diverse enough, these models might miss some key factors that contribute to risky areas, which is something that needs to be addressed.
10. **Community Outreach Needs Improvement:** Despite improvements in analytical tools for traffic safety, community engagement and outreach are still relatively low. This suggests a real need to develop programs that educate people about traffic safety and encourage their involvement in traffic safety initiatives.
Rochester's High-Risk Intersections Analysis of 2024 Traffic Accident Hotspots - Historical Crash Data Analysis Shapes 2024 Road Safety Strategies
The concerning rise in traffic accidents nationwide has spurred a renewed focus on road safety strategies in 2024. Recognizing the importance of understanding past patterns, cities are increasingly relying on historical crash data analysis to inform their approach. While traditional methods remain relevant, more advanced data-driven techniques, such as machine learning and multistep predictive models, are being employed to pinpoint accident hotspots. These methods are enhancing our ability to identify the underlying causes of crashes and predict future risks. Further, geographical information system (GIS) mapping allows for a clear visual representation of accident-prone areas, assisting in the targeted deployment of safety measures. However, it's imperative to acknowledge that the success of these approaches is dependent on high-quality, unbiased data. Furthermore, it is vital for community members and city officials to collaborate and act upon these insights if we want to see sustained improvements in traffic safety. In the long run, leveraging the lessons learned from historical crash data is key to the development of robust solutions that prioritize road safety and the well-being of all road users.
Road safety remains a challenge despite ongoing research and investments, with roughly 40,000 traffic fatalities annually in the US. Efforts like the 2023 National Roadway Safety Strategy aim to reduce these numbers, but the problem is complex and persistent. Distraction, for example, played a part in a notable percentage of fatal crashes in 2021, showing the variety of factors contributing to these accidents.
Modern approaches to traffic safety are shifting away from just relying on past accident data and simple statistics. We now have powerful tools, like risk maps and star ratings, which allow us to better pinpoint high-risk areas based on crash data. Further, researchers are increasingly incorporating machine learning into their analysis, hoping to find new insights and patterns in the data. A global database called IRTAD has been collecting crash data from over 35 countries since 1988, giving us a broader perspective on road safety trends worldwide and the effectiveness of various safety measures.
A comprehensive review of data-driven methods in road safety research reveals that our understanding of accidents is constantly changing and more sophisticated techniques are needed. Geospatial analysis helps us analyze the location and patterns of accidents over time, giving planners insights that can help with future safety initiatives. The goal of all these new efforts is to move beyond just reacting to accidents and instead try to address the underlying issues that contribute to them. The challenge for future research will be to continue to build on these new strategies, develop new tools, and enhance existing approaches to improve road safety and save lives.
It's clear that accident patterns and risk factors are becoming better understood through these methods. It's worth noting that factors like driver behavior, road design, and even weather play significant roles in crashes. These aspects, in addition to the use of advanced analytical techniques, are crucial to consider when designing safer streets. While these developments in traffic safety research are encouraging, it's vital to also acknowledge the limitations of these techniques. For example, models using machine learning can be skewed if the data they are trained on is inaccurate or has biases. Furthermore, despite the growing use of sophisticated data analysis, engaging the community and educating drivers on traffic safety remains a crucial area for improvement. The hope is that by combining robust data analysis, a deeper understanding of crash factors, and community outreach, we can find ways to decrease accidents and make roads safer for everyone.
Urban Planning Made Simple: AI-Powered Solutions for Smarter Cities and Sustainable Development (Get started for free)
More Posts from urbanplanadvisor.com: