Data-Driven Analysis Impact of Segregated Cycle Facilities on Urban Traffic Flow in 2024
Data-Driven Analysis Impact of Segregated Cycle Facilities on Urban Traffic Flow in 2024 - Data Collection Methods for Optimal Cycling Network Design
Gathering data effectively is becoming increasingly important for designing the best possible cycling networks in urban areas. This is especially true as cities aim to improve cycling infrastructure and accommodate growing numbers of cyclists. Having well-structured data collection systems lays the groundwork for developing robust cycling plans. Cities like Melbourne demonstrate how crucial it is to have a clear understanding of what data is needed to make well-informed decisions about bike paths and routes.
The broader picture of urban transportation is also relevant. We need to consider cycling networks within the entire transportation system, which includes cars, pedestrians, and public transit. This multi-modal approach offers a more comprehensive understanding of how changes in one mode affect others.
However, while data-driven approaches are gaining traction, there's still a gap in the underlying theory of how to design effective cycling networks. This lack of a firm theoretical base means that we need creative data-driven strategies to find the most impactful improvements. It's essential to focus on making strategic improvements rather than simply trying to improve the network randomly or through the bare minimum of investment. In short, using data to improve cycling infrastructure efficiency is a growing priority in urban planning to deal with the unique complexities of integrating bicycles into existing traffic patterns.
1. Gathering GPS data directly from cyclists offers a dynamic view of their chosen routes. This allows us to identify popular paths, but also pinpoint underused sections of the network, hinting at areas where improvements could boost usage. However, relying solely on volunteered data may introduce bias towards certain types of cyclists.
2. Cycling-centric mobile applications offer insights into the temporal patterns of bike usage, revealing peak hours and providing valuable information for designing infrastructure that can effectively handle fluctuating demand. It remains crucial to ensure the data's privacy and anonymization to protect user identities.
3. Sensor technology offers a precise means of quantifying cycling traffic, including speed and volume. This data can be particularly insightful when analyzing the impact of dedicated cycling lanes and identifying where they have succeeded in improving flow and safety, though careful calibration of sensors to accurately capture different cycling styles is needed.
4. It's intriguing to observe that many cyclists rely on smartphones for navigation. This generates a rich dataset of location information which can potentially be leveraged to design more user-friendly and intuitive cycle networks. However, the reliance on digital navigation could also limit exploration and discovery of less obvious routes.
5. Surveys of cyclists often reveal a disconnect between current infrastructure and user preferences. This is a valuable indication of areas ripe for improvement that could enhance cyclist satisfaction and, crucially, safety. However, biases in survey methodology and sample size can affect the representativeness of the findings.
6. Sophisticated simulation models allow urban planners to evaluate the potential impacts of proposed cycling infrastructure changes before physical implementation. These models can predict how the changes affect the broader urban traffic network, showing the potential benefits of enhanced cycling facilities. Nevertheless, these models rely on a range of assumptions that can affect their accuracy.
7. Examining historical cycling trends, considering factors like demographic changes and urban growth patterns, can help us anticipate future demand and adapt infrastructure accordingly. However, predicting future trends with absolute certainty remains challenging given the dynamic nature of urban life.
8. Deploying video analytics in high-traffic cycling locations offers a valuable method for capturing precise cyclist counts and understanding cyclist behavior. This quantitative information strengthens the justification for investment in infrastructure. However, concerns around privacy and data interpretation require thoughtful consideration.
9. By studying cycling patterns in cities with varying levels of cycling infrastructure, we can see quantifiable differences in urban mobility. Often, greater investment in separated cycling facilities is associated with a noticeable increase in cycling uptake. Yet, it's important to remember that other factors can influence these patterns, requiring careful analysis to determine causality.
10. It's crucial to recognize that relying on a single data source can provide an incomplete picture of cycling dynamics. For instance, prioritizing only traffic counts can neglect equally crucial aspects such as cyclist comfort and perceived safety. A holistic approach integrating multiple data collection methods is essential for making informed decisions about cycling infrastructure improvements.
Data-Driven Analysis Impact of Segregated Cycle Facilities on Urban Traffic Flow in 2024 - Impact of Segregated Cycle Lanes on Motorized Traffic Patterns
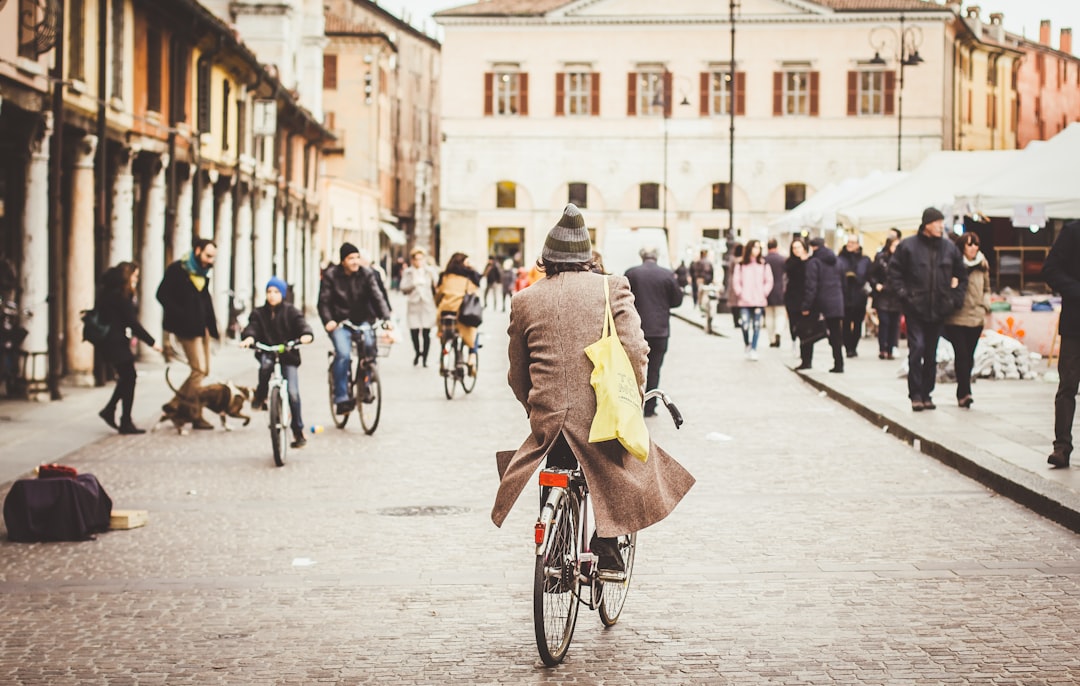
The introduction of segregated cycle lanes has demonstrably influenced the movement of motorized vehicles within urban environments. Studies examining London's Cycle Superhighway program revealed how dedicated bike lanes can alter traffic patterns, specifically impacting vehicle speeds and overall flow. Although cycling currently accounts for a relatively small percentage of trips, urban initiatives focused on increasing cycling rates, coupled with safety measures like lower speed limits, inevitably lead to changes in how motorized traffic behaves. Interestingly, research also indicates that the presence of cyclists can contribute to congestion, suggesting a complex interplay between cycling and other transport modes. This highlights the crucial role of careful urban planning, which must consider these intertwined relationships to optimize transportation systems and achieve a balance between promoting cycling and maintaining smooth interactions with motorized vehicles. While aiming to increase cycling is a positive goal, the impact on other modes of transport cannot be ignored in the design of a city's infrastructure.
The implementation of segregated cycle lanes has demonstrably influenced both cycling and motorized traffic patterns, as seen in the London Cycle Superhighways project between 2014 and 2019. Analyzing data from before and after these installations reveals shifts in traffic flow. For example, reallocating road space for cycling during the COVID-19 pandemic, as observed in comparisons of 2019 and 2021 data, showed impacts on vehicle speeds.
While cycling currently makes up only a small percentage of trips in London (around 25%), the Mayor’s strategy aims to significantly increase its share, possibly up to 5% by 2026. However, safety is a key concern discouraging wider cycling adoption. Initiatives like lowering speed limits to 20 mph have been implemented to try and address safety issues, with the goal of reducing injuries for cyclists and potentially reducing motor vehicle speeds as well.
Research suggests that, while separated lanes can promote cycling and reduce some vehicle speeds, they don’t always reduce overall congestion. The presence of cyclists can sometimes slow down motor vehicles, which leads to questions about their overall effect on the speed of traffic without dedicated infrastructure. It's important to consider that dedicated cycling networks, if built well, could improve both cycling rates and overall traffic flow.
The NITC's research into the effectiveness of lightly segregated cycle lanes points towards the need to carefully design and implement these lanes to get the desired effects. Properly enforced restrictions on illegal parking and the creation of adequate lane widths are crucial to prevent cars from interfering with cyclists.
Considering the complex interplay of vehicles and cycling patterns, we can draw parallels to emerging autonomous vehicle infrastructure. Researchers have proposed complex traffic flow models that can analyze the impact of dedicated automated vehicle lanes, and this type of rigorous analysis is useful for planning future cycle infrastructure as well. It highlights the need for sophisticated analysis when implementing changes in urban transportation patterns.
Ultimately, a data-driven approach is needed to design cycling networks that best integrate into a city's broader transportation system. This includes understanding how these changes might affect public transport schedules, freight delivery, and overall traffic flow. It is also crucial to continually evaluate how different cycling infrastructure features affect a city's evolving patterns of cycling, vehicle use, and related accident rates, including trends related to emerging transportation methods.
Data-Driven Analysis Impact of Segregated Cycle Facilities on Urban Traffic Flow in 2024 - Near-Real-Time Speed Data Analysis for Congestion Management
Near-real-time speed data analysis has emerged as a crucial tool for managing urban congestion effectively. The availability of powerful computing resources now makes it possible to analyze traffic flow in near-real-time, allowing for a more dynamic understanding of traffic patterns. This capability enables planners to pinpoint specific road sections causing congestion and to develop more refined predictions of traffic flow.
Leveraging techniques like machine learning and artificial intelligence, urban planners can harness the insights gained from real-time speed data to optimize traffic management strategies. This approach holds potential for mitigating congestion, curbing fuel consumption and pollution, and thereby promoting urban sustainability.
However, it's important to acknowledge the intricate nature of urban traffic flows. The implementation of automated systems and complex algorithms requires meticulous care. Solutions need to be designed not only to be efficient but also to ensure fairness and equitable access for all who use the urban transportation network. There's a delicate balance between implementing novel solutions and ensuring that they do not create new or worsen existing transportation inequalities.
Analyzing traffic speeds in near real-time helps us pinpoint congested areas and quickly adjust things like traffic light timings or reroute traffic. This dynamic response is crucial for keeping traffic flowing smoothly in constantly changing urban landscapes.
The use of artificial intelligence in speed data analysis is becoming more common. This allows for predictive modeling that could anticipate congestion before it happens, giving us the ability to plan ahead instead of just reacting to it.
It’s interesting that even a small drop in average car speed, like just 1 mph, can lead to a significantly lower chance of a serious accident. This underlines the importance of using updated traffic control strategies to manage congestion and prioritize safety.
The detailed nature of real-time speed data lets us differentiate between various types of traffic, such as delivery trucks, buses, and regular cars. This level of detail is helpful in identifying what's causing the biggest congestion problems, allowing us to focus on solutions that address those specific issues.
We're finding that people's travel behavior during rush hour follows certain patterns. For example, we see speed increases on some roads just before the typical end of the workday, which tells us that we need traffic management strategies that can handle those types of shifts in traffic.
One challenge with using real-time speed data is making sure it's accurate and reliable. This is particularly true when collecting data from multiple sensors spread across different parts of the city. We need a consistent way of calibrating these sensors to make sure we get dependable data.
It’s interesting to see how the location of bike lanes can impact not just cycling-related traffic but also the flow of nearby car traffic. For instance, adaptive lane management systems that support mixed modes of transport can potentially benefit both cyclists and drivers.
Real-time speed monitoring is great for spotting impacts from outside factors like weather or major city events. This allows transport agencies to make temporary changes to traffic management in order to handle congestion more effectively when it pops up unexpectedly.
Comparing speed patterns before and after implementing cycling infrastructure helps us identify areas where the system might not be running as efficiently as possible. This can lead to opportunities to change infrastructure or policies to create a more efficient transport network in urban environments.
Integrating more advanced telematics and connected vehicle technologies has the potential to greatly improve near real-time speed data analysis. This could pave the way for smoother integration of cycling infrastructure into the overall traffic system.
Data-Driven Analysis Impact of Segregated Cycle Facilities on Urban Traffic Flow in 2024 - Deep Learning Models for Short-Term Urban Traffic Flow Prediction
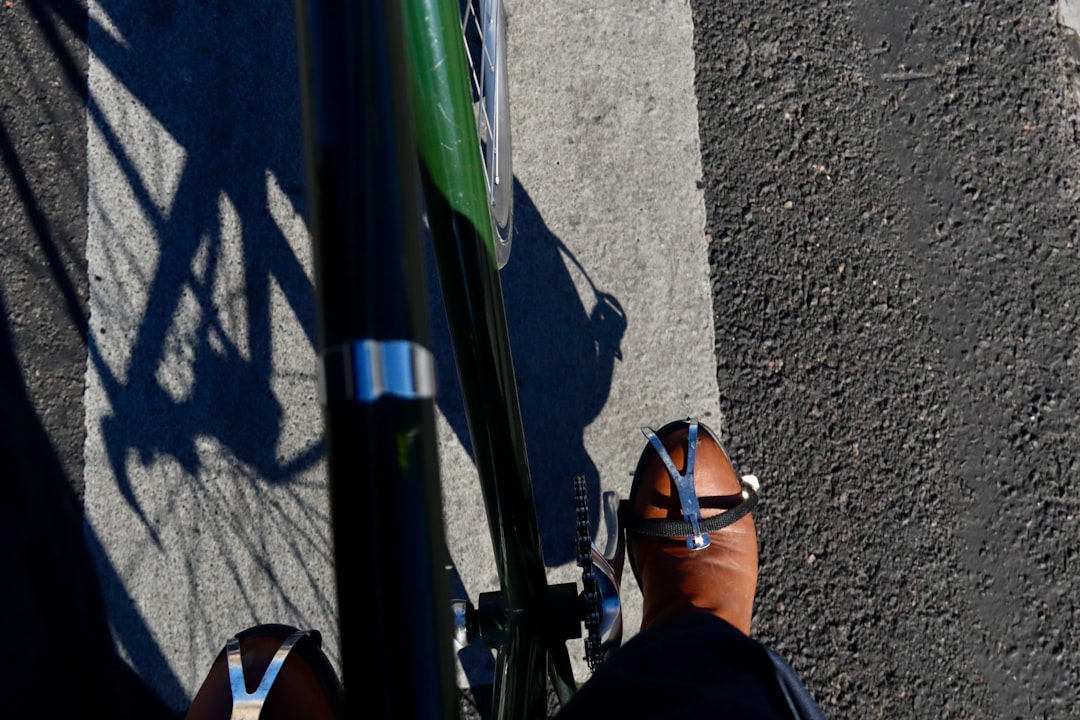
Deep learning has emerged as a powerful tool for predicting short-term urban traffic flow, surpassing the limitations of traditional methods. Previously, predicting traffic flow often relied on simpler machine learning techniques, which struggle to handle the unpredictable nature of urban environments. Accidents, construction, and weather can significantly disrupt traffic patterns, creating challenges for these simpler models. Deep learning approaches, like the CDLP model, utilize a combination of different neural networks (such as convolutional neural networks and LSTMs), providing greater flexibility in adapting to the dynamic and complex nature of urban traffic. By integrating real-time data from various sources, including social media, these models can react more effectively to current traffic conditions, leading to more precise short-term predictions. The ability to accurately predict short-term traffic flow is not only helpful for managing congestion but also plays a role in designing urban transport systems that incorporate cycling infrastructure and other features in a more intelligent and effective way. While promising, these models are constantly evolving, and research is ongoing to address limitations and uncertainties in their predictions.
Predicting urban traffic flow over short periods is increasingly relying on deep learning models, particularly those using recurrent neural networks (RNNs). These models can capture intricate temporal patterns in the data that more traditional methods often miss, potentially leading to more accurate predictions. However, the selection of features for these models is crucial. Including things like weather, time of day, or special events in the data can make predictions more precise by adding relevant context. Unlike simpler models like linear regression, deep learning approaches automatically find relationships between variables, which can reveal intricate connections between diverse traffic elements that are challenging to model otherwise.
Despite their advantages, deep learning models need considerable computing resources for training, which is a concern for smaller cities or situations where computing resources are limited. It can be difficult to scale them up for real-time applications. Understanding exactly why a deep learning model makes a certain prediction is also an ongoing challenge. While these models can offer very accurate forecasts, interpreting the reasoning behind the predictions can be complex, making it harder for urban planners and policymakers to apply them effectively.
Specialized RNNs, such as Long Short-Term Memory (LSTM) models, are particularly good at handling long-term relationships in time series data. This makes them well-suited for traffic flow prediction where historical traffic patterns have a significant impact on future flow. Integrating deep learning with the growing network of Internet of Things (IoT) devices like traffic cameras and sensors allows a continuous stream of real-time data into these models, making the predictions even more adaptable and accurate.
To make these models more robust, researchers are exploring techniques like adversarial training. These methods aim to improve the reliability of deep learning models in the face of unusual data patterns or unpredictable events. This is important for making sure that traffic flow forecasts remain helpful even when things don't follow their usual patterns. Recently, transfer learning has emerged as a promising method for improving deep learning model performance when data is limited. The idea is that urban planners can use models that were already trained on traffic datasets from other cities to improve predictions in new or data-poor environments.
A common issue with deep learning models is the tendency to overfit the training data. If the dataset is too small or not diverse enough, the model can become too closely tied to the specific data it was trained on, resulting in poor predictions on new, unseen data. It's therefore crucial to use strategies like cross-validation and model regularization to ensure that these sophisticated methods remain effective for accurate prediction over time. This involves testing how well the model performs on data it wasn't specifically trained on, which helps prevent it from becoming too specific to just one particular dataset.
Data-Driven Analysis Impact of Segregated Cycle Facilities on Urban Traffic Flow in 2024 - Visualization Techniques for Complex Traffic Trajectory Analysis
Visualizing complex traffic trajectory data has become increasingly important for comprehending the intricate behaviors of vehicles and cyclists within urban environments. These techniques translate raw data into understandable visual representations, allowing planners to detect patterns and trends hidden within large datasets. Interactive visualization tools, for instance, facilitate the exploration of traffic movements across space and time, providing deeper insights into lane-changing behaviors and interactions between different road users. In the context of data-driven urban planning, especially when evaluating the impacts of segregated cycle facilities, effective visualization is crucial for informed decision-making. This emphasis on visual analysis reflects a larger trend in using data to tackle the challenges of managing urban transportation, striving to achieve more efficient, safe, and sustainable transportation networks. However, the effectiveness of these techniques can sometimes be limited if the visual representations are not thoughtfully designed, which is an ongoing challenge in this field. It's vital to ensure the methods are used to support, but not replace, comprehensive analysis and interpretation of data.
1. Visualizing traffic trajectory data with heat maps can reveal hidden congestion points that may not be obvious from standard traffic data. This can provide a fresh perspective on the intricate movement of vehicles within cities. It's intriguing how these visualizations can uncover unexpected patterns of congestion.
2. The ability to visualize traffic data in real time allows traffic engineers to swiftly adjust traffic signal timing in response to changing patterns. This dynamic approach has the potential to significantly enhance traffic efficiency and alleviate congestion. It would be interesting to see how this could impact existing traffic control systems.
3. Using tools like clustered scatter plots can visually illustrate the relationships between cycling activity and other factors, such as road quality and nearby land use. This kind of visual analysis can provide urban planners with more complete insights when making design choices. However, establishing causality is still an area requiring careful consideration.
4. 3D visualizations are powerful tools for understanding multi-layered traffic flow dynamics, especially in complex environments like intersections. This can help us develop infrastructure designs that are more attuned to the intricate ways vehicles and cyclists interact in these areas. This has the potential to greatly improve the understanding of how different transportation systems interact at junctions.
5. Integrating time-related information into visualizations allows us to create animations that show how traffic patterns change over time, such as hourly or seasonally. This can help us time maintenance work or infrastructure upgrades more effectively. However, it is important to remember that any predictions of future patterns are contingent on various assumptions.
6. Interactive dashboards allow urban planners and other stakeholders to explore different scenarios, including what might happen if we build new bike lanes. This kind of analysis can help us better understand the influence that changes to cycling infrastructure might have on overall traffic behavior. While promising, it’s important to validate these simulations with real-world data for accuracy.
7. Data visualizations can reveal how adding dedicated bike lanes affects not only cyclist safety but also the movement of pedestrians in the surrounding area. It becomes very clear that the different transportation modes in a city are deeply intertwined. However, understanding these connections in a way that is actionable and helps us improve safety for all users remains a challenge.
8. Using clear and understandable visualizations can help facilitate communication with the general public on the challenges and benefits of developing better cycling infrastructure. By involving citizens in these processes, we can promote greater buy-in for infrastructure improvements. However, challenges can arise in how we translate technical insights into broadly understood presentations.
9. Machine learning is enhancing the capabilities of visualization. Rather than just presenting static snapshots, visualizations can now reveal dynamic trends. This more detailed analysis transforms raw data into actionable insights for urban planning. However, ensuring the accuracy of these models remains a crucial issue.
10. Augmented reality (AR) can be used to help engineers and urban planners visualize proposed modifications to the urban environment before they are actually built. This approach offers a more intuitive way to evaluate the potential impact of such projects. However, the complexity of the underlying algorithms for AR and the quality of underlying traffic data can be factors in the effectiveness of the representations.
Data-Driven Analysis Impact of Segregated Cycle Facilities on Urban Traffic Flow in 2024 - Adapting Cycling Infrastructure to Multiplex Transport Networks
In the pursuit of sustainable urban environments, integrating cycling infrastructure into complex transport networks is gaining significant attention. This multifaceted approach acknowledges the need to seamlessly weave bike paths into the existing fabric of urban mobility, including cars, buses, and rail systems. Urban planners are increasingly employing data-driven methodologies to dissect intricate travel behaviors and traffic flow patterns, which ultimately guides the creation of efficient and interconnected cycling networks. Yet, the process faces challenges in harmonizing new cycling infrastructure with existing urban layouts, especially since cycling networks often develop at a slower pace compared to established transit systems. A comprehensive view that recognizes cycling as an integral part of a wider urban transport ecosystem is crucial for cultivating a more balanced and sustainable future for cities. This includes acknowledging that simply adding bike lanes is not always sufficient and the wider implications need to be fully considered. There is a need to avoid making the mistake of viewing this as just a bike path issue and instead, seeing it as a vital element within a larger interconnected urban transport system.
Urban areas have historically prioritized car traffic, leading to a significant portion of city space being dedicated to roads. We can conceptualize a city's street network as a layered system, a multiplex transport network, with different layers representing modes like bikes, buses, and trains. To effectively plan cycling infrastructure, data-driven methods are critical. They help with routing decisions and expanding the cycling network.
Recent advancements in analyzing cycling environments utilize shared bike trip data, providing insights into cyclist behavior and their perceived cycling conditions. This multi-modal approach, considering various transportation modes together, is becoming increasingly crucial for making urban mobility more sustainable and efficient, especially in major cities. Viewing transportation through the lens of network science, where each mode interacts as a layer in a larger system, gives a more holistic perspective.
However, often, bicycle networks develop in a piecemeal manner, without the comprehensive planning seen in other areas of urban transport. Analyzing traffic data across all modes provides valuable insights into how individuals travel and the overall system's performance. Studying the effect of dedicated cycling lanes on traffic flow is important for making informed choices in the complexity of urban transportation.
Developing a robust, data-driven system would facilitate the discovery of important factors that improve the cycling experience and, ultimately, the whole urban transportation infrastructure. A deeper understanding of how cyclists interact with the overall system is needed for better design. It's also worth noting that the specific needs and preferences of cyclists aren't always uniform; it's possible that improvements in infrastructure might lead to more bike traffic, which could potentially negatively impact other modes of transportation. However, carefully considered designs for bike lanes could enhance both cycling and overall traffic flow. This nuanced perspective is vital when evaluating the effects of dedicated cycle lanes on the intricate network of urban transport.
More Posts from urbanplanadvisor.com: