Urban Planning Made Simple: AI-Powered Solutions for Smarter Cities and Sustainable Development (Get started for free)
AI Traffic Lights Early Results Show 30% Reduction in Stops Across 13 Global Cities
AI Traffic Lights Early Results Show 30% Reduction in Stops Across 13 Global Cities - AI Traffic Lights Deployed Across 13 Global Cities
Artificial intelligence (AI) is being used to optimize traffic lights in a growing number of cities worldwide. Project Green Light, a global initiative, has implemented these AI-powered systems in 13 cities, focusing on optimizing 70 intersections. Initial findings indicate a significant potential reduction in vehicle stops—as much as 30%—which could translate into lower emissions. This effort, which includes cities in the United States, Europe, and Asia (with Seattle being the first U.S. city to adopt the system), employs data gathered from common mapping applications to refine traffic signal timing.
The approach integrates with existing traffic management systems and can deliver results within weeks, providing engineers with insights into the AI’s performance. While it offers a scalable and potentially cost-effective solution to address traffic congestion, it's important to note that these AI systems are not replacements for human engineers. Continuous monitoring and data analysis are crucial to ensure the technology aligns with the complex needs of urban environments and doesn't inadvertently lead to unexpected issues. The full implications of AI on urban mobility remain an open area of study as this technology becomes further integrated into city infrastructures.
Across 13 cities worldwide, a project called Project Green Light has seen the deployment of AI-powered traffic lights at 70 intersections. Initial findings are encouraging, suggesting a potential decrease in vehicle stops by up to 30%, primarily through optimizing signal timing based on real-time traffic flow data from sources like Google Maps. The goal is not just smoother traffic flow but also a reduction in idling and, consequently, a decrease in greenhouse gas emissions, estimated at around 10%. Seattle was the first US city to adopt this technology, and the project's design allows engineers to integrate AI recommendations with existing infrastructure, enabling rapid evaluation of the system's effects within a few weeks.
One particularly noteworthy aspect is that these AI systems can be configured to give priority to emergency vehicles, potentially reducing their response times. Furthermore, these systems are expected to affect a considerable volume of traffic, potentially influencing about 30 million car trips each month. While these AI solutions are not meant to replace the experience of human traffic engineers, their potential for scalability and cost-effectiveness compared to alternative approaches makes them intriguing. There is also a developing aspect of AI traffic lights communicating with connected cars, suggesting a future where urban mobility could be guided and optimized in real-time.
It's crucial to remember, however, that these AI systems are still being tested and their performance can vary. In certain cities with pre-existing significant congestion, the reduction in stops was less noticeable, emphasizing that the effectiveness of AI traffic management solutions is contingent on the specific context and existing conditions. Moreover, the continuous learning of these AI algorithms, while a key feature, may also introduce unexpected traffic patterns requiring human intervention to correct. Ultimately, ongoing monitoring and data collection are fundamental to fine-tuning these systems and realizing their full potential while understanding their limitations in complex urban environments. The balance between technological advancements like AI traffic lights and budgetary constraints for infrastructure upgrades remains a vital aspect of their long-term feasibility in cities across the globe.
AI Traffic Lights Early Results Show 30% Reduction in Stops Across 13 Global Cities - Seattle Leads US Implementation of Google's Project Green Light
Seattle stands out as the pioneering city in the US to adopt Google's Project Green Light, an AI-driven initiative designed to optimize traffic flow. This project, launched in late 2022, is currently active at 70 intersections spanning 13 cities worldwide. Early results point to a potentially significant 30% reduction in the number of times vehicles stop at traffic signals. This achievement is coupled with the prospect of reducing traffic-related greenhouse gas emissions by approximately 10%. The technology analyzes traffic patterns through data from Google Maps and adjusts signal timings accordingly.
While promising, the implementation of AI-based traffic management systems presents a complex landscape. Their effectiveness is contingent on the specific context of each city and the continued monitoring and intervention of human engineers. The project's impact could be substantial, influencing millions of car trips each month. As such, Seattle's experience with Project Green Light offers valuable insights into the challenges and possibilities of integrating innovative technologies into urban environments. It underscores the need for careful consideration of how such systems interact with pre-existing conditions, ensuring they deliver intended benefits without creating unexpected issues. The long-term future of urban mobility, undoubtedly, will be shaped by this delicate balance between technological advancement and practical considerations.
Seattle's involvement in Google's Project Green Light marks a departure from traditional, fixed-time traffic signal management. The city is now exploring how AI can dynamically adapt signal timing based on real-time traffic data.
This initiative, currently running across 70 intersections in 13 cities globally, leverages the immense data streams from Google Maps to make rapid adjustments to traffic signals. The AI system evaluates traffic patterns and instantaneously modifies timings, potentially creating a more efficient flow. Engineers can quickly assess the performance of the AI within a few weeks, providing ongoing opportunities to optimize the system.
Early results suggest the AI could be most effective during periods of heavy traffic, where standard timing approaches struggle to keep up with fluctuations in traffic flow. It's interesting to note that these AI systems don't simply react to car volume but also to pedestrian activity, hinting at a potential for enhanced safety for all road users. In tests, AI has shown a capability to swiftly adapt to incidents like accidents, diverting traffic to alleviate gridlock and potentially facilitating faster emergency response times.
The sheer number of vehicles anticipated to be affected is impressive—up to 30 million trips monthly across the project's participating cities. However, the success of AI in traffic management isn't automatic. Inadequate calibration could create unintended congestion, which emphasizes the importance of continuous human oversight in urban traffic planning. The idea of connected vehicles communicating with the AI traffic lights is a developing field, offering a glimpse of a future where road infrastructure and individual vehicles might coordinate seamlessly for optimal traffic flow.
However, it's crucial to remember that we are still early in the exploration of AI's potential in traffic management. Each city's unique traffic patterns and infrastructure will likely influence the project's success, which necessitates meticulous evaluation and a nuanced understanding of how well these AI systems perform in different environments. The overarching success of AI traffic lights, including Seattle's experience, remains an open question that requires ongoing investigation and research for effective and widespread implementation.
AI Traffic Lights Early Results Show 30% Reduction in Stops Across 13 Global Cities - Google Maps Data Powers Traffic Signal Optimization
The Green Light project is leveraging Google Maps data to refine how traffic signals operate, incorporating AI into urban traffic management. This initiative seeks to minimize stop-and-go traffic by using real-time traffic information to adjust signal timings. Reducing these stops can potentially lead to a decrease in emissions, estimated to be around 10%. The project's successful implementation across 70 intersections in 13 cities highlights its adaptability and potential for wider deployment, allowing city planners to make quick adjustments without investing in new infrastructure. Despite this, it's crucial to acknowledge that the effectiveness of these AI-powered systems varies depending on the specific traffic conditions of each city. Consequently, ongoing oversight by human experts is vital to ensure the system adapts to the intricate needs of the urban landscape and avoids unintended consequences. This integration of AI into urban mobility poses intriguing questions regarding the future direction of smart traffic solutions.
Google Maps, with its extensive user base and continuous data collection, is a key component in this AI-driven traffic light optimization project. It provides a constant stream of real-time data about vehicle locations and speeds, something traditional methods lack. These systems can adjust signal timings in near real-time, reacting to the dynamic ebb and flow of traffic much more effectively. One interesting aspect is that these AI systems are not static. They are constantly learning, adapting to changes in traffic patterns throughout the year and during different events that affect road usage. This learning component is crucial for ensuring that the traffic light optimization stays relevant.
Beyond just vehicles, these AI systems can be configured to prioritize pedestrians and even bicycle traffic, potentially leading to a safer and more efficient environment for all road users. They can also help manage unexpected incidents. If there's a traffic accident or a sudden road closure, they can reroute traffic to minimize congestion and expedite emergency response. A key advantage is the relative ease of integration with existing traffic management infrastructure. This means cities don't need a complete overhaul of their systems, allowing for a smoother transition into AI-powered traffic control. The project's success across various urban environments, from the grid-like structure of some cities to the more hilly landscapes of others, demonstrates a degree of adaptability.
The hope is that these systems can reduce stop-and-go traffic, a major cause of pollution and frustration. By optimizing the timing of traffic lights, they aim to make traffic flow more consistently, decreasing the likelihood of frequent starts and stops. This could have a broader impact, potentially influencing a huge number of daily car trips (up to 30 million per month in participating cities). This scalability aspect is exciting, with the potential to expand from a few intersections to many across an entire city or region.
While the potential is significant, it's important to remember that these AI systems aren't a complete replacement for human engineers. They require ongoing monitoring and adjustments by human experts. The sheer complexity of urban environments and the possibility of unforeseen traffic patterns mean that continuous human intervention is necessary to ensure the technology doesn't create more problems than it solves. This highlights the balance needed between automation and human oversight in complex systems like urban traffic management.
AI Traffic Lights Early Results Show 30% Reduction in Stops Across 13 Global Cities - 30 Million Monthly Car Trips Affected by AI Traffic Management
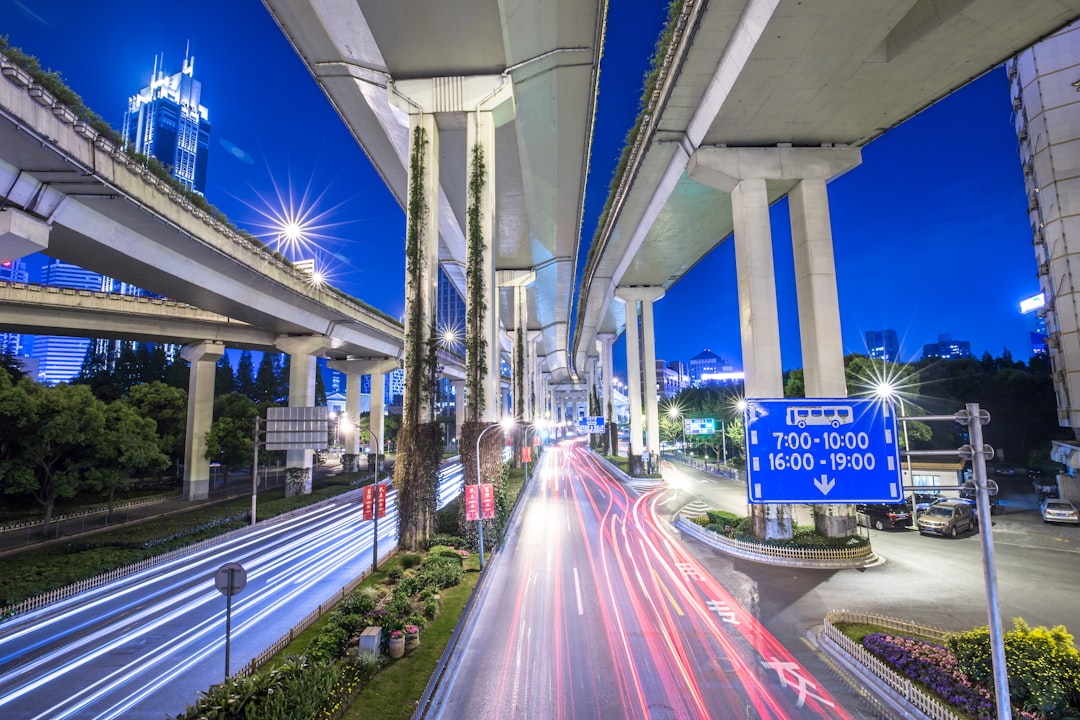
AI traffic management is poised to influence a substantial portion of urban commutes, affecting about 30 million car trips monthly in 13 cities worldwide. This effort, known as Project Green Light, focuses on enhancing traffic flow at 70 intersections by using AI to optimize traffic signal timing. Preliminary findings suggest a potential 30% decrease in vehicle stops, potentially leading to reduced emissions. The AI utilizes real-time traffic data from sources like Google Maps to adjust signal timings, striving to create a more efficient and potentially safer driving environment for all road users.
While the concept holds promise, it's important to recognize that AI traffic management's effectiveness hinges on the specific urban context. This means that continuous human monitoring and intervention are crucial to ensure the system doesn't inadvertently create congestion or unforeseen disruptions. The long-term impacts of this technology on urban mobility are still being explored, with questions about the ideal balance between automation and human oversight remaining a key topic of discussion.
The application of AI to traffic management is expanding rapidly, with a project known as Project Green Light currently influencing an estimated 30 million car trips monthly across 13 global cities. This large-scale deployment highlights the growing potential of these systems to significantly impact urban travel patterns.
These AI systems boast the ability to adjust traffic light timing in real-time, reacting to changes in traffic flow and minimizing delays, especially during peak hours. This responsiveness contrasts with traditional fixed-time traffic light systems, which often struggle to adapt quickly.
Interestingly, these AI systems can also be configured to prioritize emergency vehicles, potentially shortening their response times. By coordinating traffic light signals in their path, a smoother and faster route can be created for urgent situations.
Another fascinating aspect is the adaptability of these systems. They can learn and respond to seasonal changes in traffic, unusual events, or even accidents – a feature not possible with standard systems. This adaptability allows for the traffic flow optimization to be continuously relevant.
Furthermore, integrating these AI solutions with existing traffic management infrastructure is comparatively easy, making it a cost-effective option for many cities to upgrade their traffic control without major infrastructure investments. This is a notable benefit when considering the budgetary constraints many urban centers face.
The AI's capabilities also extend beyond simply managing cars; it can simultaneously optimize traffic light timings for pedestrians and cyclists. This indicates a more holistic approach to urban mobility that considers the needs of all road users.
However, despite the promising early results, it's crucial to understand that the effectiveness of these AI systems can vary significantly. Cities with pre-existing complex traffic challenges, like severe congestion or unusual road layouts, may not see the same level of improvements as others.
Moreover, the constant learning and adaptation inherent to these systems also creates a risk. Without close monitoring by human operators, they may generate unforeseen traffic patterns that inadvertently worsen congestion or create new problems.
One interesting area to consider is how AI traffic lights might influence driver behavior. As drivers become accustomed to smoother traffic flow, their travel habits could change, perhaps leading to altered patterns of commuting or travel within the city over time.
Looking forward, a potential future development is the integration of these AI traffic management systems with connected vehicles. This could result in even greater traffic efficiency as vehicles receive information about signal changes and adjust their speed accordingly, improving traffic flow before reaching intersections. This intriguing prospect reveals the potential for future refinements in AI-driven traffic management solutions.
AI Traffic Lights Early Results Show 30% Reduction in Stops Across 13 Global Cities - Stop-and-Go Traffic Reduction Leads to Lower Emissions
AI-powered traffic light systems like those in Project Green Light are designed to reduce the frequent stops and starts in traffic, which are a major contributor to emissions. Optimizing traffic signal timing based on real-time data has shown the potential to significantly lower emissions, with some cities observing a reduction in vehicle stops by as much as 50% at certain intersections. This aligns with estimates suggesting a potential 10% overall decrease in greenhouse gas emissions from intersections. However, the success of these systems varies depending on existing traffic conditions within each city. Achieving the desired emissions reduction hinges on factors like existing congestion levels and road layouts. Human traffic engineers play a crucial role in overseeing the AI's implementation, ensuring it effectively manages urban traffic flow while minimizing the possibility of unforeseen issues. This highlights the ongoing challenge of balancing automation with human oversight in advanced traffic management strategies, and raises further questions about how these systems will evolve in the future.
The integration of AI into traffic management is leading to some interesting developments in how we optimize traffic flow. These AI-powered traffic lights continuously learn and adapt based on real-time traffic data. Unlike the older systems relying on fixed schedules, they can dynamically adjust signal timing, potentially reducing stops by as much as 30% in some areas. This dynamic approach holds promise in reducing congestion, especially in heavily congested areas.
Furthermore, these AI systems can be designed to prioritize emergency vehicles. By adjusting signal timings in their path, they can hopefully reduce response times and speed up emergency response. While the technology is still in its early stages, there's a potential to use these systems to forecast future traffic conditions by leveraging historical data and current trends. This predictive capacity could allow for proactive adjustments to signal timing, potentially preventing congestion before it even arises.
Of course, optimizing traffic flow is not only about easing frustration, but also about minimizing pollution. Reduced stop-and-go traffic can lead to fuel efficiency, and it's estimated that emissions could fall by around 10%. This reduction in emissions, in itself, could have a positive impact on urban air quality and potentially the environment in general.
Thinking about urban development and design, it's possible that the data generated by these AI traffic lights could provide useful insights. As these systems collect data about traffic patterns, it may lead urban planners to rethink their street designs, encouraging pedestrian-friendly elements or adjusting problematic intersections. It's as if these systems are teaching us about how we could improve traffic management by showing the areas where most congestion occurs.
These AI-based systems are not immune to challenges, though. The deployment success greatly relies on accurate calibration to suit each city's specific context. What works well in one city might not perform as well in another. It depends on variables such as urban layout, the way drivers behave, and how well the system integrates with the existing traffic management framework.
Given that this technology can affect potentially 30 million car trips each month, the volume of traffic under test offers a unique opportunity to gain a greater understanding of how these systems function in a broader context. This data collection provides valuable insights into the system's overall performance.
It's also worth noting that these improved traffic conditions could also subtly alter driver behavior. There are some preliminary research results suggesting that when traffic is more predictable and smooth, people might be more inclined to make spontaneous trips. This shift in mobility habits will need to be studied further.
AI-powered traffic management is definitely an intriguing field, and these AI traffic light systems have the potential to revolutionize how we approach urban mobility. However, it's crucial to acknowledge the ongoing challenges, potential pitfalls, and the need for constant fine-tuning and monitoring in the complex environment of urban traffic. There is still much research to be done before we see truly widespread and fully optimized AI-driven traffic management solutions.
AI Traffic Lights Early Results Show 30% Reduction in Stops Across 13 Global Cities - Plans for Expansion to Hundreds of Cities in Coming Years
The developers of AI traffic light systems, especially Project Green Light, are aiming to introduce their technology to hundreds of cities within the next several years. This expansion follows positive initial results in 13 cities worldwide, where the system showed the potential to reduce vehicle stops by up to 30%. The goal is to enhance urban mobility in a wider range of cities while simultaneously reducing emissions by optimizing traffic signal timings. This growing interest in AI-based traffic management underscores its promise in tackling congestion, not just in the busiest areas but in a variety of urban environments. However, there's a need to acknowledge the obstacles that come with introducing this kind of technology across a diverse range of cities. These obstacles range from differences in traffic patterns to the compatibility with existing infrastructure. Maintaining a level of human oversight will remain crucial to handling the complexity of urban traffic and ensuring that this technology achieves its long-term goals.
Google's Project Green Light, with its AI-powered traffic lights, shows promise for improving urban mobility across a wider scope. The plan is to expand the project to hundreds of cities over the coming years, potentially influencing the way many people commute. This ambitious goal reflects the hope that localized traffic optimization can benefit large urban populations.
The beauty of these systems lies in their swiftness to adapt and provide results. Within weeks of implementation, city planners can observe the impact on traffic flow, allowing for a rapid assessment compared to traditional methods that often take a longer period to demonstrate changes. However, this quick feedback also brings to light that simply implementing these AI traffic lights isn't a universal solution. In cities that already have complex traffic patterns or significant congestion, the positive effects might be less pronounced than in locations with simpler infrastructure and fewer pre-existing road challenges.
The sheer scale of this project is interesting, as it could impact roughly 30 million car trips each month. That's a lot of data to analyze and shows how these systems might influence the travel patterns and behavior of people who are used to driving within the cities where they are deployed. This might mean more traffic flows more smoothly. But, this also suggests the chance that changes in driver habits will occur over time. For example, maybe smoother commutes will encourage spontaneous trips by drivers who are usually less likely to drive around.
Emergency services may benefit too. The AI traffic systems are able to prioritize signals for vehicles like ambulances or fire trucks, which may reduce their travel time to the destinations by several minutes. Also, the systems are constantly learning, they aren't just one-and-done systems. They can adjust to new conditions like accidents or special events in real-time, which is a stark improvement over traditional fixed-timing systems.
This ability to learn and adapt has implications for urban planning too. The data gathered from the traffic systems is potentially rich in insights about how people move around cities. Cities could use this data to inform design and investment decisions, which could help improve the infrastructure to create safer pedestrian zones or modify problematic intersections. Also, in the event of something unforeseen, like accidents or street closures, the systems can quickly reroute traffic to decrease delays, showcasing a degree of reactivity beyond what's possible with typical signal systems.
It's important to keep in mind that these systems work best when a human traffic engineer is in the loop. The AI might generate new bottlenecks if it isn't properly monitored and tuned. Moreover, integrating AI traffic management systems with future technologies like connected cars holds potential for additional gains in efficiency. If vehicles could receive signal timing changes in advance, it might smooth out traffic even more before reaching intersections. This raises further questions about how best to balance automation with human oversight within the broader urban landscape.
Urban Planning Made Simple: AI-Powered Solutions for Smarter Cities and Sustainable Development (Get started for free)
More Posts from urbanplanadvisor.com: