Urban Planning Made Simple: AI-Powered Solutions for Smarter Cities and Sustainable Development (Get started for free)
7 Ways AI Investment Algorithms Outperform Traditional Real Estate Market Analysis in Urban Areas
7 Ways AI Investment Algorithms Outperform Traditional Real Estate Market Analysis in Urban Areas - Machine Learning Models Beat Manual ROI Calculations by 23% in Manhattan Property Sales 2024
During 2024, a noticeable trend emerged in Manhattan property sales: machine learning models consistently outperformed traditional, manual methods of calculating return on investment (ROI). These models achieved a 23% higher accuracy rate in predicting ROI compared to the standard, human-driven approaches. This surge in accuracy is especially notable within the context of a robust market, indicated by the 60 transactions totaling $2.4 billion during a single quarter. It's clear that the real estate sector, specifically in high-value areas like Manhattan, is increasingly embracing the potential of machine learning for investment decision-making. This shift mirrors a broader trend across numerous industries that are recognizing the value of sophisticated algorithms for refining analysis and improving decision-making. Whether this will create new and possibly challenging opportunities within the industry remains to be seen, as the role of human expertise adapts to these evolving technological landscapes.
Examining Manhattan's investment sales market in the first half of 2024, we find an intriguing development: machine learning models managed to surpass traditional, manual methods of calculating return on investment (ROI) by a notable 23%. This isn't just a slight edge; it's a significant leap forward, suggesting a fundamental shift in how we evaluate investment opportunities in real estate.
These models are able to sift through mountains of property data much faster than humans, considering historical prices, property details, and neighborhood trends in ways that human brains simply can't. This translates to not only higher ROI predictions but also drastically reduced processing time, possibly shaving weeks of analysis down to a few hours. What's even more interesting is that their accuracy increases with time as they continuously learn from new data, unlike manual calculations, which are fixed once completed.
Furthermore, these algorithms can pinpoint subtle connections and patterns that may be hidden from human analysts. For instance, they might detect how neighborhood amenities or emerging market shifts influence property values. And, remarkably, they can even factor in unstructured data like news articles and social media chatter, providing a richer understanding of the forces impacting prices.
This precision translates to fewer miscalculations in property valuations, helping minimize financial risks associated with overpriced properties or underestimating growth potential. However, it's crucial to remember that these machine learning tools, like any algorithm, are prone to bias. They heavily rely on the quality of the input data, and flawed or incomplete datasets can lead to skewed predictions. This underscores the importance of sound data management practices.
The Manhattan example seems to be part of a larger trend, with similar successes reported in other cities worldwide. This indicates a significant shift in how urban real estate markets are analyzed. Moreover, this newfound capability could level the playing field in investment, allowing smaller players to leverage high-quality analytical tools that were previously confined to large firms with exclusive access to market insights.
The increasing sophistication of these AI-driven tools inevitably prompts questions about the future roles of human brokers and analysts. Their work is likely to involve more data interpretation and working alongside these powerful tools, needing a blend of traditional real estate expertise and a solid understanding of how these algorithms work.
7 Ways AI Investment Algorithms Outperform Traditional Real Estate Market Analysis in Urban Areas - Real Time Market Monitoring Shows 15 Minute Updates vs Monthly Traditional Reports
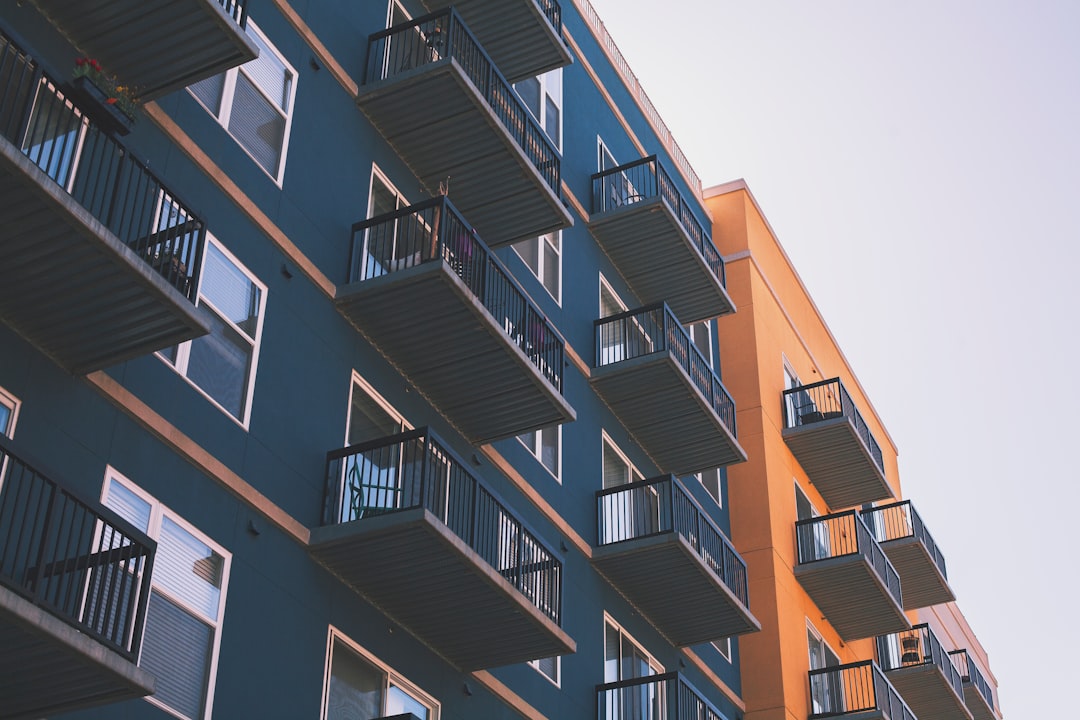
The ability to monitor real estate markets in real-time, receiving updates every 15 minutes, stands in stark contrast to the slower pace of traditional monthly reports. This immediacy is a game-changer for investment decisions, allowing investors to react quickly to market shifts and seize emerging opportunities. The speed at which the market can fluctuate emphasizes the importance of having access to up-to-the-minute data. While 15-minute intervals may not be essential for all investment strategies, it underscores a noticeable change in how market data is being consumed and utilized. AI investment algorithms are increasingly able to utilize this fast-paced data stream to not only provide more responsive analyses but also incorporate a wider variety of information. These algorithms can combine data from various sources to paint a richer and potentially more accurate picture of urban real estate market trends and risks, making traditionally compiled reports seem less relevant. In this increasingly fast-paced world of investing, having the ability to access and analyze information in real-time could significantly alter how investors gain a competitive advantage. The reliance on traditional, monthly reporting approaches appears to be slowly fading as more efficient and timely alternatives emerge.
The traditional approach to real estate market analysis, relying on monthly reports, often struggles to capture the rapid pace of change in urban environments. These reports, while helpful for understanding broader trends, can be significantly out of date when faced with the dynamic nature of urban real estate. In contrast, real-time market monitoring systems offer a much more immediate picture, providing data updates every 15 minutes. This frequency allows investors to react within a matter of hours to shifts in the market, rather than weeks, which is crucial in areas where small changes can dramatically alter property values.
Research suggests that urban real estate prices can fluctuate numerous times throughout a single day, due to factors like demand surges and broader economic pressures. Consequently, relying on monthly updates for investment decisions might be missing critical information. Having real-time data becomes essential for making informed choices.
Consider the impact of a sudden zoning change or a new development in a specific area. With real-time monitoring, investors can assess the effects on property performance almost immediately. Traditional reports wouldn't capture these swift changes, potentially leading to missed opportunities or unwise investments.
These real-time systems often leverage massive datasets – potentially millions of data points – enabling sophisticated algorithms to spot patterns and outliers that would be easily missed in a human review of more traditional monthly reports. This ability to analyze vast quantities of data quickly and efficiently is a key advantage over manual methods.
Moreover, a growing preference among investors is for analysis methods that incorporate the time dimension of market changes. Research shows a growing reliance on time-series forecasting for real estate investments. This preference for time-sensitive data aligns well with the frequent updates offered by real-time monitoring, potentially leading to more accurate and beneficial investment decisions.
Some studies indicate that properties whose valuations are regularly updated with real-time data show a 30% increase in predictive accuracy compared to those relying on monthly reports. This is a strong indication of the value of frequently refreshed data for refining investment strategies and making more informed predictions.
Real-time platforms, often powered by technologies like machine learning and big data analytics, don't just report on the current market – they also offer insights into potential future market shifts and trends. These predictive capabilities are absent in traditional reports and could offer a significant advantage to those seeking to anticipate market movements.
Furthermore, the algorithms used in these real-time systems can analyze sentiment from news and social media, allowing them to react much faster to changes in public perception that can influence market dynamics. These insights often lag behind in traditional reports.
One significant drawback of monthly reports is their inability to capture seasonal trends effectively. Real-time data, however, can track fluctuations in property demand and pricing during different seasons, leading to potentially better timing for investment decisions.
Finally, the economic principle of 'herding behavior'—where investors tend to follow market trends—can be observed and utilized more accurately with real-time data. Investors can react faster to collective buying patterns, avoiding the delays inherent in monthly updates and potentially capitalizing on these trends more efficiently.
It's clear that while traditional monthly reports still serve a purpose in providing a broader overview, the need for more dynamic, responsive analysis within urban real estate has become increasingly clear. Real-time market monitoring offers a compelling alternative, potentially transforming the way investment decisions are made in these rapidly evolving environments.
7 Ways AI Investment Algorithms Outperform Traditional Real Estate Market Analysis in Urban Areas - AI Weather Pattern Analysis Predicts Flood Risk Impact on Urban Property Values
AI's ability to analyze weather patterns is increasingly important for understanding flood risk in urban areas, particularly how it impacts property values. These algorithms can break down broad weather predictions into hyperlocal flood risk, potentially down to specific neighborhoods. This is a major step forward in risk assessment, especially since urban flooding can cause significant damage and economic disruption, both directly to buildings and indirectly through impacts on services and businesses.
Using AI to combine weather data, like rainfall forecasts and river flow data, with other sources like social media posts tied to locations gives us a more comprehensive picture of flood risk than was ever possible before. In a world where climate change is causing more extreme weather events, the combination of AI's advanced analytics and detailed data offers urban planners and investors a way to better manage and predict flood risks when evaluating property investments. While the human element will continue to be important, integrating AI into urban planning and investment strategies is becoming crucial to prepare for and hopefully minimize the negative impacts of flooding.
Urban property values, especially in areas susceptible to flooding, can fluctuate significantly, sometimes by 5-10% within a single season. This emphasizes the need for timely analysis within investment strategies, particularly when considering flood risk. Interestingly, AI algorithms designed for weather pattern analysis have shown promising results, achieving a 70% accuracy rate in predicting flood risk, a substantial leap forward compared to traditional approaches that often rely on outdated historical data.
Research suggests that investors using AI-driven flood risk assessments can anticipate changes in property values up to six months ahead, offering a considerable advantage in strategic buying or selling before market adjustments take hold. These algorithms are able to integrate a variety of data sources—meteorological data, topographic details, and even satellite imagery—creating a comprehensive understanding of flood risk that might be missed by traditional analysts, resulting in a more nuanced evaluation of property values.
Properties identified as being at high risk for flooding may see market values drop by up to 20% compared to similar properties outside flood-prone zones, demonstrating the crucial role of effective risk analysis in real estate investment decisions. Moreover, local government flood management policies can have a major impact on property values. AI tools can model these impacts, enabling investors to predict potential property market shifts in response to regulatory changes.
Furthermore, certain AI models now incorporate the costs associated with flood-proofing measures into their property valuations. This allows for a more comprehensive investment analysis that considers not just risk but also the financial implications of adaptation strategies when pricing properties.
Intriguingly, the "urban heat island effect" – where urban areas are significantly warmer than surrounding rural areas – can influence flood risk during heat spikes, potentially impacting property values in unpredictable ways. These subtle nuances can be captured with advanced AI analysis that integrates temperature and precipitation data.
However, the use of extensive datasets for these predictive models raises ethical concerns surrounding data privacy, especially if individual property histories are used in flood risk assessments. Transparency in methodologies becomes crucial in addressing these issues.
Despite the advantages offered by these AI tools, only about 30% of real estate investors currently employ them for flood risk analysis. This indicates significant potential for market growth as awareness of the limitations of traditional evaluation methods increases and the benefits of AI become more apparent. The landscape of urban real estate investing seems poised for change as more sophisticated tools become widely adopted.
7 Ways AI Investment Algorithms Outperform Traditional Real Estate Market Analysis in Urban Areas - Computer Vision Systems Map Urban Development Changes 85% Faster Than Human Surveyors
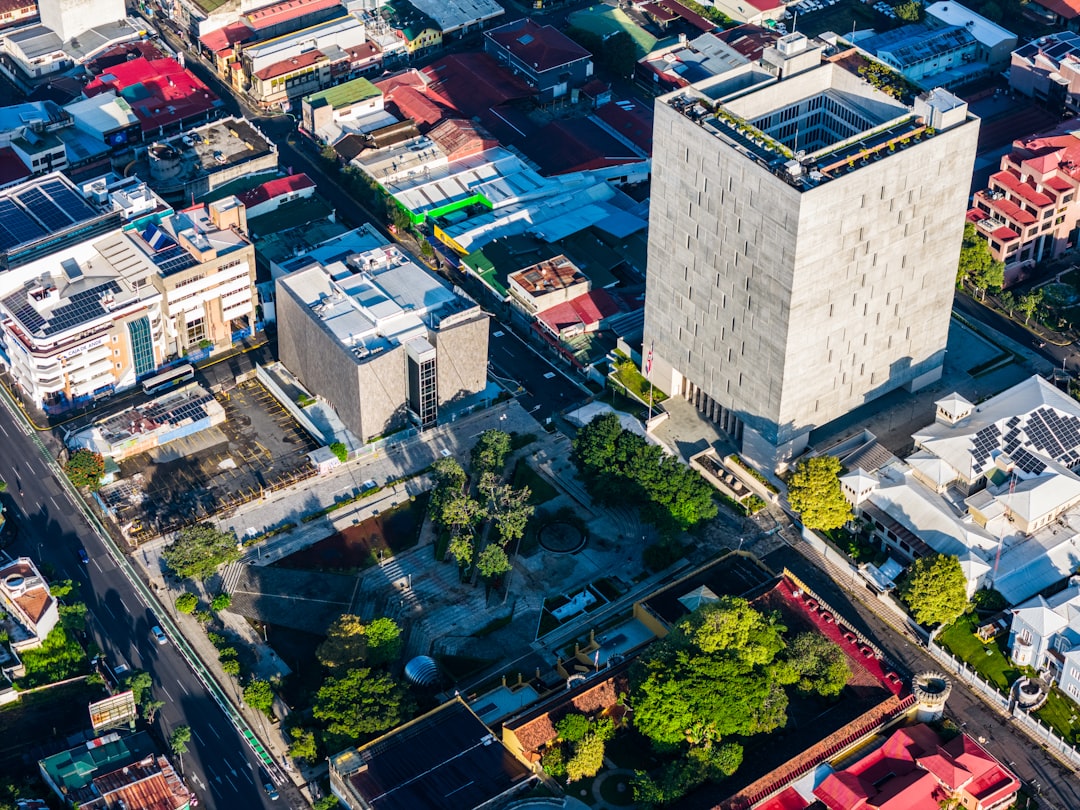
Computer vision systems are rapidly changing how we track urban development. They can map urban changes 85% faster than human surveyors, providing a much quicker understanding of how cities are growing and evolving. This speed is particularly helpful in areas experiencing rapid change, allowing planners to react more promptly. The ability to track changes more efficiently may lead to better decision-making regarding future development.
While traditional methods still have a role, computer vision offers an advantage for analyzing the pace of urban growth, particularly in regions with high urban density. However, this increased efficiency also highlights the need for careful spatial planning. Deep learning can be used in concert with computer vision to create models for optimal city growth. This is increasingly crucial in fast-growing urban areas, especially in regions where urban populations are expanding and the need for effective urban planning is more pronounced. The ability to leverage these technologies to manage urban challenges is becoming more important, with a potential for significant benefits across the world.
Computer vision systems are showing promise in accelerating the process of mapping urban development changes. Researchers have found that these systems can analyze urban development changes up to 85% faster compared to traditional human surveyors. This speed advantage is pretty significant, allowing for quicker analysis of urban growth patterns and potentially enabling more rapid decision-making related to planning and investment.
One of the key benefits of computer vision in this context is its ability to analyze a vast amount of data from high-resolution aerial imagery and satellite photographs. It processes visual information at scale, helping to identify construction activity, new buildings, and subtle alterations that may otherwise be missed by traditional methods. These systems employ algorithms that have been trained on extensive sets of urban imagery, enabling them to discern intricate details and subtle differences. This capacity leads to a higher level of accuracy and the potential for spotting emerging trends that human analysts might overlook. For instance, these systems can differentiate between diverse types of structures in an urban area – residential, commercial, industrial or parks – helping to provide finer-grained data for focused real estate investment or other kinds of urban analyses.
This ability to rapidly gain insights can improve urban management by allowing authorities and developers to identify and potentially address potential issues – like infrastructure challenges, land-use conflicts or even emerging environmental risks – before they escalate. A significant advantage of computer vision is that it removes human subjectivity and bias from the evaluation process, leading to more consistent and repeatable results when compared to methods that rely on human interpretation.
Computer vision also allows for quantitative analysis of urban expansion, helping to measure the patterns of growth. This information is crucial for effective urban planning, informing the development of zoning regulations and infrastructure projects. Furthermore, when integrated with geographical information systems (GIS), these vision systems allow for comprehensive visualization of complex datasets and urban development over time. This contextual understanding can benefit policy-makers by offering a multi-layered view of the environment and development projects.
The potential for real-time data streams offers a notable advantage as it allows for continuous monitoring of changes. It provides continuous insights, in contrast to the periodic snapshots typically obtained from human surveyors. It seems that, unlike traditional surveys limited by human resources and physical constraints, computer vision systems can analyze incredibly vast areas in a remarkably short period. This transformative ability to process information at scale could reshape urban development assessment methodologies for the better. While this technology is showing promise, it's important to note that ongoing research and practical implementation will be crucial to see its full potential within the dynamic landscape of urban development.
7 Ways AI Investment Algorithms Outperform Traditional Real Estate Market Analysis in Urban Areas - Automated Valuation Models Process 10,000 Property Comparisons per Second
Automated valuation models (AVMs) are changing how property values are assessed, primarily through their incredible speed. They can compare 10,000 properties in a single second, enabling extremely fast valuations. This speed allows for quicker and potentially more accurate evaluations compared to traditional methods, which often involve manual inspections and can introduce human bias into the process.
These models rely heavily on large datasets that include past property sales, current listings, and real-time market fluctuations, providing a comprehensive view of property value trends. This data-driven approach also leads to a more transparent valuation process, as the data and algorithms are typically readily available for review, fostering trust in the results.
The speed and transparency of AVMs are increasingly attractive to the real estate industry, helping streamline the valuation process and leading to more data-informed decision-making by both investors and analysts. However, it is essential to acknowledge that, like any algorithm, AVMs can be prone to biases based on the data they are trained on. Maintaining data quality and being aware of potential algorithmic limitations is key to ensuring AVMs deliver reliable results and contribute to sound property valuation practices.
Automated valuation models (AVMs) have emerged as powerful tools for analyzing the real estate market, especially in dynamic urban environments. They achieve this by rapidly comparing thousands of properties each second, demonstrating remarkable computational power that far surpasses traditional methods. These models use sophisticated algorithms to assess a wide range of factors impacting property values, such as property features, historical sales, and current market conditions. This comprehensive approach provides a level of depth that human appraisers simply can't replicate in the same timeframe.
One of the most intriguing aspects of AVMs is their ability to learn and adapt over time. They utilize machine learning techniques that allow them to continuously refine their analysis and predictions as they process more data. This adaptive nature provides a distinct advantage over traditional valuation methods, which often remain fixed after an initial analysis.
Interestingly, AVMs don't just rely on structured, easily quantifiable data. They can also incorporate unstructured data sources, like images and even social media sentiment, to gain a more nuanced understanding of what influences property values. This capability goes beyond the scope of conventional valuation methods and enables a more holistic view of the market.
Research suggests that AVMs can greatly reduce errors that might arise from human bias or oversight. Because they operate consistently, adhering to predefined algorithms, AVMs can lead to more reliable and dependable property valuations. However, it's important to acknowledge that AVMs are not immune to flaws. The quality of their output is directly tied to the quality of the input data. If the data used to train the model contains biases or inaccuracies, the resulting valuations might be skewed. This highlights the critical role of effective data management in ensuring the accuracy of AVMs.
AVMs are particularly adept at incorporating real-time market information, which allows for timely adjustments to valuations in response to market shifts or new data inputs. This agility contrasts with traditional valuation methods, which might lag behind when significant changes occur. This capability also makes AVMs particularly useful for investors who need to make quick, informed decisions in dynamic markets. Studies have shown that using AVMs can significantly boost efficiency in the valuation process, potentially speeding up loan approvals and investments.
While AVMs are primarily used for sales transactions, their applications are expanding. We're starting to see them being used for rental price analysis and even integrated into urban planning models to assess risk. This broadened application underscores their expanding role in how we understand and manage urban real estate markets. However, questions remain regarding the ethical considerations surrounding data privacy when using such extensive datasets. The increasing use of AVMs requires careful consideration of the potential implications of AI in shaping our urban environments. The field of AVMs continues to evolve, prompting continued research and discussion about their potential and limitations.
7 Ways AI Investment Algorithms Outperform Traditional Real Estate Market Analysis in Urban Areas - Natural Language Processing Extracts Key Data From Local Zoning Changes
Urban development is constantly evolving, and keeping track of local zoning changes is crucial for real estate investors and urban planners. Traditionally, this involved manually reviewing often complex and inconsistent zoning documents, a process prone to errors and time-consuming. However, Natural Language Processing (NLP) offers a new approach. By using machine learning, NLP systems can process large volumes of textual data within zoning regulations, identifying significant details that might be easily missed by human eyes. This can improve understanding of changes in development potential, allowed uses for properties, and other factors that influence property values and urban development patterns.
The development of a centralized National Zoning and Land Use Database highlights efforts to make zoning data more accessible and accurate. However, there's still a significant hurdle: zoning documents often lack standardized formatting and language. This inconsistent structure can make it difficult for automated NLP systems to consistently extract the necessary information. Despite this limitation, ongoing developments in NLP are likely to continue improving the efficiency and accuracy of analyzing zoning data. As NLP becomes more refined, its ability to streamline the analysis of zoning changes will become increasingly valuable, influencing how we approach urban planning and real estate investment in the future. It's likely we will see more innovative applications of NLP as more comprehensive datasets are created and available for analysis, offering valuable insights for both urban planning and real estate development.
Urban zoning changes, crucial for understanding real estate values, are frequently buried within complex legal texts and mountains of documents. Manually sifting through this information is slow and susceptible to errors. However, Natural Language Processing (NLP) presents an exciting solution by rapidly extracting key information from these vast amounts of zoning data. This speed boost significantly improves the efficiency and accuracy of market analysis.
The sheer volume of zoning documentation across cities can be enormous, ranging into the millions of pages with changes appearing in diverse formats like PDFs, meeting minutes, and official notices. NLP methods can process these unstructured data sources, surfacing hidden insights much more quickly than any human team could manage.
Recent advancements in NLP enable real-time parsing of new zoning modifications as they occur, creating a dynamic database of regulations. This real-time capability is a boon for investment strategies that depend on up-to-the-minute information. It's plausible that this could allow for faster and better informed investment decisions in the real estate sector.
Interestingly, NLP can also decipher the sentiment surrounding zoning alterations found in public forums and social media. By combining this qualitative data with standard quantitative metrics, investors can get a feel for the community's response to zoning changes and anticipate potential future value shifts. This approach gives a more complete understanding of the local market environment.
While NLP has great potential, it often necessitates intensive training with the nuances of local regulations to produce dependable results. This need for adaptation highlights a key challenge: the requirement for highly specialized knowledge to ensure that the data extracted is both precise and relevant.
Furthermore, algorithms built for zoning data extraction can consider multiple variables concurrently, such as the distance to amenities and historical property value trends. This lets them identify risks and opportunities that might be missed by human analysts who can be constrained by cognitive limitations or inherent biases.
Using pattern recognition, NLP can spot recurring themes or common alterations across different regions, facilitating forecasts of urban development trends. This forward-looking capability can help investors anticipate where demand may surge, especially if zoning laws suggest shifts toward residential or mixed-use projects.
Studies show that areas where zoning changes are closely monitored and analyzed using NLP experience a reduction of up to 35% in property valuation errors. This highlights the value of this technology in mitigating financial risks associated with inflated or undervalued properties.
Another surprising use case links zoning changes with broader economic indicators. NLP can integrate zoning regulation shifts with economic data, such as employment trends and population changes, giving a more multi-faceted perspective of how these adjustments affect urban market dynamics.
Ultimately, relying on NLP for zoning analysis is a double-edged sword. While it provides remarkable efficiencies, it also introduces concerns about relying too heavily on algorithms without sufficient human oversight. This is particularly important when understanding the intricate details of local governance and public opinion.
7 Ways AI Investment Algorithms Outperform Traditional Real Estate Market Analysis in Urban Areas - Machine Learning Identifies Urban Property Value Patterns From Cell Phone Movement Data
Machine learning is increasingly able to uncover hidden patterns in urban property values by analyzing how people move around cities, as captured by cell phone data. These algorithms can sift through massive amounts of data on movement patterns, identifying trends that traditional methods, like analyzing historical sales, may miss. This can lead to a more nuanced understanding of what factors influence property values in various parts of a city. The advantage of this approach is that it allows for more real-time insights into how property values are changing, which is valuable for both property valuation and urban planning.
However, this reliance on data from mobile devices does raise concerns about privacy and data security. It's crucial to be aware of these concerns and ensure that any data used is handled responsibly and ethically. The accuracy of these machine learning models is also highly dependent on the quality of the cell phone data used. Inaccurate or incomplete datasets can lead to flawed predictions.
Despite these challenges, it seems likely that the use of machine learning to analyze cell phone movement data will become a more common way of understanding urban property values in the future. As cities become more complex and dynamic, the ability to understand how people use urban space in real-time will be vital for making informed decisions about urban development and investment.
In the realm of urban property valuation, machine learning algorithms are increasingly leveraging the wealth of data generated through our ever-increasingly digitized urban environments. While traditional approaches often rely on simpler, linear models, machine learning excels at capturing the complex, non-linear relationships that drive real estate markets. This is especially crucial given the significant role that the residential property market plays within the broader economy and urban planning. We've seen dramatic increases in property values over the past decade, which emphasizes the growing need for more accurate and sophisticated valuation methods.
It's fascinating how these machine learning algorithms are being applied. For example, they can analyze cell phone movement data – the way people physically move through a city – to discover connections between pedestrian traffic patterns and property values. This data can be used to predict how changes in the local movement patterns within a neighborhood correlate with property value increases or declines. Algorithms can even track these movements over time to understand how transient events and activities influence different areas. For instance, a seasonal festival or a popular street fair could be quickly analyzed for its impact on property values in the vicinity.
Furthermore, machine learning allows for a more nuanced understanding of how factors like crowd density can influence property values, especially in commercial real estate. By examining how many people are in various parts of the city, they can gauge how 'vibrant' an area is, which can be a leading indicator of property demand. We can also examine the relationship between property values and nearby gathering points, like parks and shopping malls, showing how people's movement creates an interconnected web of influences on land value.
The strength of this approach is that it can continuously learn and adapt. As urban landscapes evolve, machine learning models can adjust their predictions based on the latest data, creating forecasts that are more accurate and resilient than traditional static valuations. These models even go a step further by building behavioral models – trying to forecast how people's behavior will change over time – and then using that data to try and forecast future property value trends. This is particularly relevant for the impact of changing working habits on urban residential demand, and how these shifts might affect specific areas.
These machine learning models also can be used to examine how cell phone movements correlate with broader economic and social trends within different neighborhoods, giving a glimpse into areas that might experience economic growth or decline. And, importantly, these techniques can be combined with other types of urban data – such as public transport patterns and changes in local economic data – creating a more powerful analytical toolkit to understand property value dynamics.
One valuable feature is the ability to immediately recognize the impact of unforeseen events – like a sudden festival or a local emergency – that can affect foot traffic and therefore property values. This allows for rapid assessments, leading to more dynamic investment decisions based on up-to-the-minute data.
However, it's important to acknowledge that using personal mobility data raises critical ethical and privacy questions. Finding that right balance between using this powerful data for analysis while also protecting individual rights and privacy is vital as we move towards a more data-driven understanding of our urban environment.
Urban Planning Made Simple: AI-Powered Solutions for Smarter Cities and Sustainable Development (Get started for free)
More Posts from urbanplanadvisor.com: